The KIB podcast
The KIB podcast is a podcast from the University Library where we tackle topics related to the scientific publishing landscape and research infrastructure. We also explore other areas a university library is passionate about.
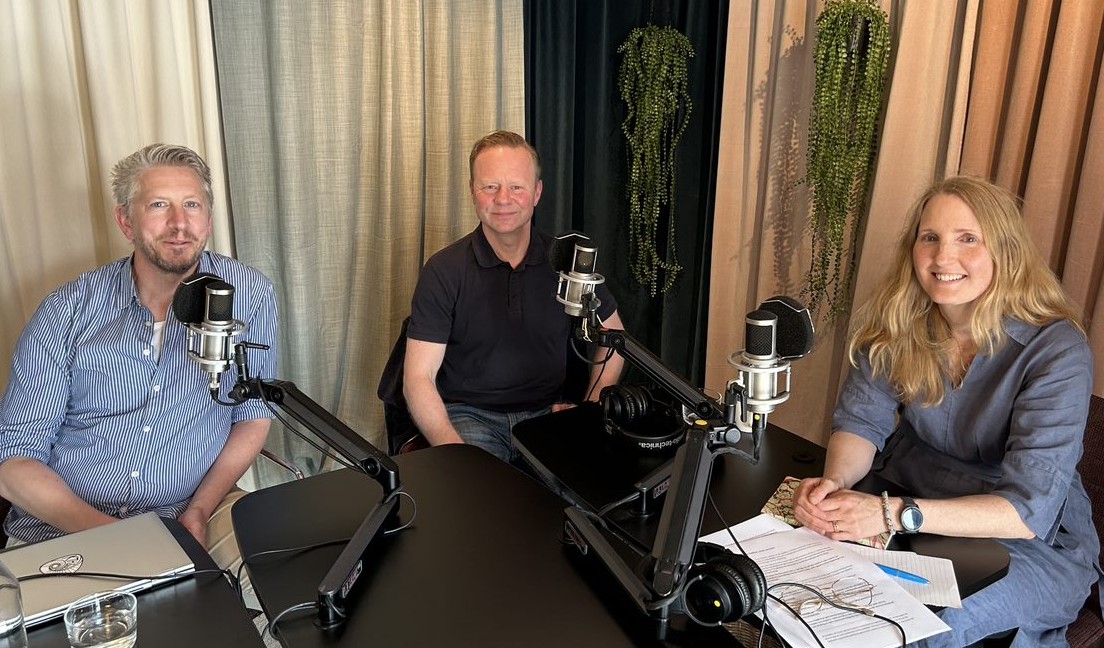
Episode 4: Open science and why it matters – the libraries, the researchers and the many aspects on open science
In this episode we take a closer look at open science, why it matters, and how it can help us meet some of the major challenges facing research today. With growing concerns about research funding, especially recent cuts in the US that may affect core scientific infrastructure, the need for openness, collaboration, and resilient research practices has never been greater.
Joining this conversation are Lina Waltin, librarian at Karolinska Institutet University Library, Patrik Magnusson, senior researcher and the chair of the Open Science Working Group at Karolinska Institutet and also the director of the Swedish Twin Registry and Lars Nordesjö, the head of publication infrastructure and media at KTH library. Together, they explore what open science means in practice, its benefits, the challenges it brings, and what the future might hold.
Special thanks also to Erik Åkesson Kågedal, librarian at Karolinska Institutet University Library for his help in providing background information for this section.
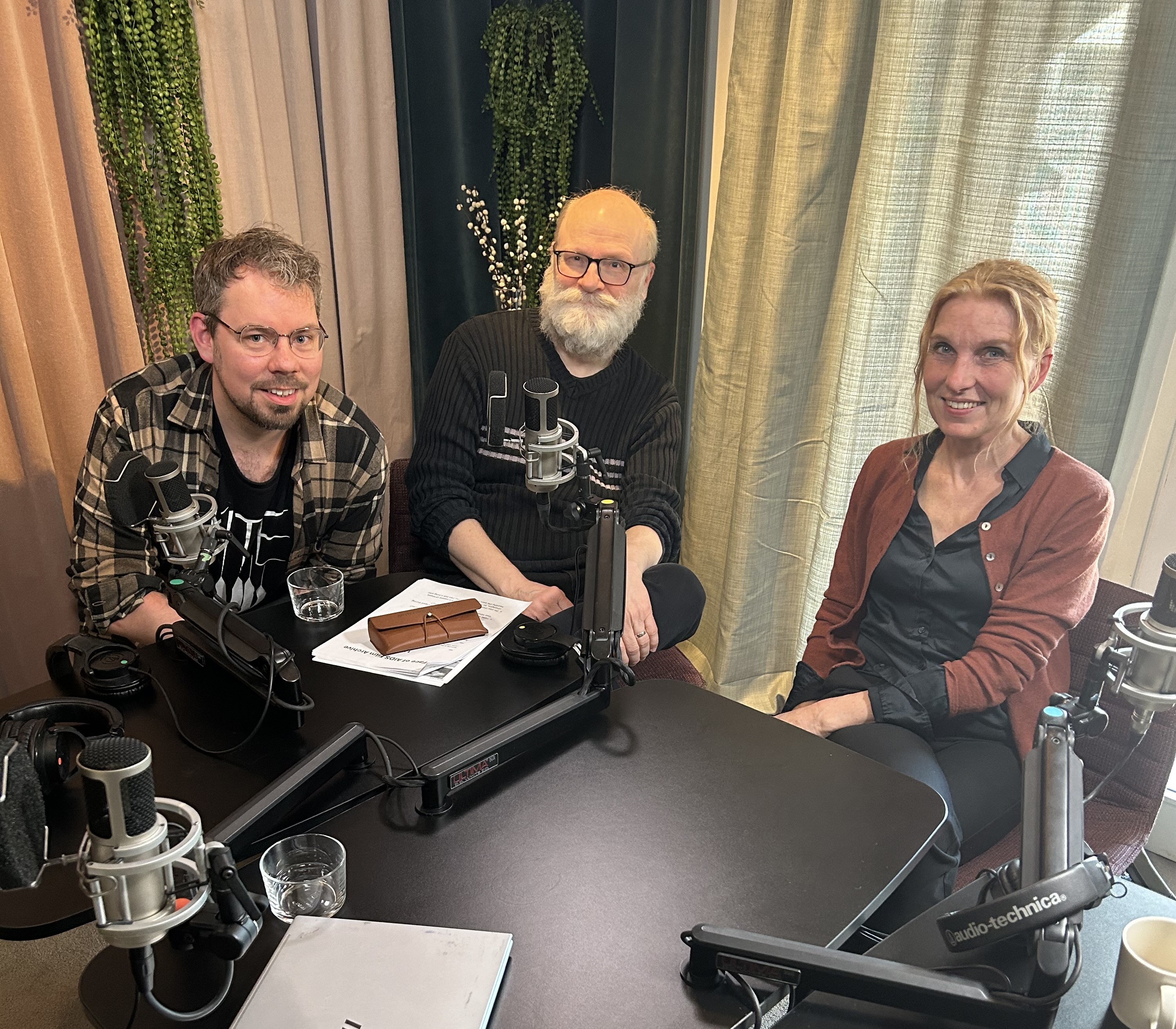
Episode 3: AIDS – yesterday, today and tomorrow
In this episode of the KIB podcast, librarians Martin Andersson and Martin Kristenson talk to Anna Mia Ekström, Professor of Global Infectious Disease Epidemiology at Karolinska Institutet. We present the Face of AIDS Film Archive, which documents the global HIV epidemic 1986-2021, and discuss what is happening now in the work against HIV and AIDS.
"One thing that struck me when I recently went through clips from the archive is the amazing commitment of the activists," says Anna Mia Ekström in the podcast. "It was young people and people living with HIV, but also mothers, researchers, doctors, Nobel laureates, monks – everyone was involved in the fight against the virus in the 80s and 90s, they really made their voices heard. We don't see that today, it's silent – and we know that silence kills."
Links and more information
Transcript of the KIB podcast episode 3
This is a transcript of the KIB podcast episode 3. The transcript is made by AI (there might be minor mistakes).
Talare 2
Welcome to the KIB podcast, the podcast from the Karolinska Institutet University Library. I'm Martin Andersson, librarian.
Talare 3
And I'm Martin Kristensson, also librarian at KI.
Talare 2
And this episode will be about the Fates of AIDS film archive, the online archive based here at the library. And today we have a special guest with us, Anna-Mia Ekström, professor in global infectious disease epidemiology at Karolinska Institute. Welcome. It's a long title.
Talare 1
Thank you. Yes.
Talare 2
We just dive straight in here. How long have you been working with HIV issues?
Talare 1
For the last 25 years.
Talare 3
And how would you say the HIV situation has changed during that time in Sweden and internationally?
Talare 1
Well, dramatically. When I started to work with HIV around year 2000, then the drugs had just really become available. in high-income countries, but we're still lacking to a large extent in most African countries that were most hardly hit also by the HIV and AIDS epidemic. So I've seen what can happen when you don't get the medicine you need to survive. And we really, I've sort of built my career or my research around hope and around seeing what remarkable difference it makes when you have access to antiretroviral treatment and also prevention. And when we also are available to reduce stigma around HIV, it makes such a It can make such an incredible difference. It's the difference between life and death, really. And I've also worked as a clinician. I work as a clinician, as an infectious disease clinician, and see patients and people living with HIV on a weekly basis. So I also have a feeling for what it could be like to be living with HIV then and today. And that's also a really positive development.
Talare 1
Right now, in 2025, we are extremely worried that we will backtrack and lose what we've gained over the last few decades due to the new US administration's severe cutbacks on HIV funding and development aid. So it's almost like the circle is closing.
Talare 3
Do you think that people in general are aware that you can live a long and healthy life with HIV?
Talare 1
Well, I think in general, a lot of people are today aware that there is medication, effective medication for HIV. They may not be aware that with these drugs, you are actually able to live a very long and healthy life with HIV without ever getting sick from the virus and never dying from AIDS, never developing AIDS, and that you're no longer at risk of transmitting. HIV to anyone else. That means undetectable equals untransmittable. So the great, great majority actually of people in the world who are on HIV medication have no detectable virus in their bodies and can't transmit it, which is absolutely amazing. I don't think everybody knows that. And I think we know less about HIV in a country like Sweden as compared to a country in Africa where they are much more aware of how HIV can affect society.
Talare 2
How should we make people more aware of these possibilities?
Talare 1
So how can we make more people more aware of these antiretroviral drugs exist, but also other? really effective preventive measures that we now call PrEP, like pre-exposure prophylaxis for HIV, which is two drugs instead of three that you get if you have the infection already. You can actually take drugs to prevent HIV from ever entering your body. So that's called PrEP. And it's also a big sort of scientific breakthrough. And I think in some Key populations, the awareness is quite high, for example, among men who have sex with men who have access to PrEP, for example, in Sweden and in many other sort of Western high-income countries. PrEP has also up to very recently been available for young women, for example, in Southern Africa that are at high risk of HIV. But in general, we talk much less about HIV today. It's not like headline news anywhere. People think it's like yesterday's disease, while in fact almost 4,000 people are still infected every day, 3,600 it's estimated. And we have more than a million people dying from AIDS, which is a totally preventable cause of death every year. So I think we need to talk about it.
Talare 1
And with the tragic developments over the last few months, We will be forced to see young people dying from AIDS again, babies becoming infected with HIV even more to a larger extent than we already see. It's about 100,000 babies get HIV from their mothers every year, down from about half a million at the peak of the AIDS epidemic. But we really fear that these numbers will go up again. it will be in our faces, the face of AIDS.
Talare 2
Yeah, and now we're just going to talk a bit more about the archive, and we'll go back to the beginning of the face of AIDS film archive. And Martin, you have worked with the face of AIDS film archive since 2013, when the entire archive was digitized and handed over to the Karolinska Institutet library.
Talare 3
Yes, and the largest part of the collection consists of film director Staffan Hildebrandt's documentation of the AIDS epidemic from 1986 to 2021. That's 35 years. And that is a lot of material, roughly 700 hours of footage. And in 2017, we launched the Phase of AIDS website, and it all went online.
Talare 2
And about 300 films are now available on? the archive's website, free to everyone to access. And you don't have to log in or register to watch this film. To get access to a large part of the archive or the full archive as a researcher or student, you can apply for an account on the archive website.
Talare 3
I should add that the archive is quite unique in that it spans over such a long time and covers about 40 countries. So in the archive, you can follow the HIV and AIDS history from the first year in the 80s when there was no medicine and an AIDS diagnosis meant an almost certain death to the situation today with effective treatment. And there are interviews in the archive with all sorts of people involved in the HIV response, not only scientists, but activists, people living with HIV, drug users, and many others from all corners of the world. And you are also free to screen the films, say, for education or public meetings, as long as you give credit to the archive. And Anna-Mia, as a researcher, what value do you see in an archive that documents an epidemic like this?
Talare 1
I think it's incredibly important because a lot of people don't remember or weren't even around at the time when AIDS really haunted the world. And I think it's so important to hear these young people's stories and life stories so that we never forget them and also don't go there again. And that's why it's so important to document what's happening in the world, not only. back then, but actually also today. And I am worried that we are not documenting enough today.
Talare 3
Have you used the face of AIDS? Are you working as a researcher or teacher?
Talare 1
Yes, I'm responsible for a course on HIVs that's been running for the last 25 years or so, where we use this material. And we also have a special sort of session. dedicated to the history of the pandemic because it's vital to really understand the stigma that is still surrounding HIV AIDS, that people still mix HIV and AIDS. And the fact that many key populations are also discriminated for other reasons are still at higher risk for HIV infection, which makes HIV still being, you know, a big deal. I work with As a clinician, I meet people who are newly diagnosed with HIV or I am the person who needs to tell them that your test is positive. And to be able to do that and still give hope to say, you know, it might, you know, you might feel horrible today or be really worried, but I can say with, you know, 100% certainty that you will, you know. live a long and healthy life. You can't transmit HIV. You will not, you know, risk of transmitting HIV to anyone else, to your partners or to any, you know, future babies.
Talare 1
And the medicine is free of charge and normally without side effects. And it's often just one tablet a day. And that's an absolutely amazing development. And then it's important to be able to see what it was like just a few decades ago when first there was nothing. And young people were dying in large numbers, you know, undermining societies, families, the whole infrastructure of these countries that were most affected. And then when the treatment came in high-income countries in the mid-90s, late 90s, and in Africa, it started to be scaled up in around 2005 and forward 10 years later, which is in itself, you know, a shame. Anyway, in the beginning, people had to take like 30 tablets a day, something like that, to survive. And today we're down to one. So that history is also important to document. The successes that research has contributed to is fantastic, especially when research today is underfunded and at threat.
Talare 3
Do you remember any specific clip in the archive as valuable or important?
Talare 1
Well, I'm particularly touched, I think, always when young people appear in these films and they're very sick. You know, it gets to you as the person touches your heart to see that and they know they're dying and they're still, you know, they want to communicate to the world what they're experiencing. And some of them are still really, you know, working as activists. And also, I think countries that I've been to and where I've worked with HIV and visited are also sort of, they're more closer to my heart and to my mind, of course, because I can relate to these contexts and these people.
Talare 2
As a researcher and a clinician, why haven't you engaged so much in this question?
Talare 1
I think because it covers everything in life that is sort of to me. It's related to sort of being a human being, not always being logical, love, sex, life and death, but also it's very closely associated with politics, gender equality or inequality rather, key populations or those, you know, most vulnerable populations that are already discriminated in our society, such as People who use drugs, people who sell sex, men who have sex with other men. It's associated with homosexuality, issues that many find very difficult to talk about. So those who were most affected by HIV early on were also groups that were already oftentimes stigmatized or discriminated upon. So it comes natural to engage. with, you know, people where you feel that you can actually make a difference, a big difference as a researcher and as a clinician. And despite the medicines, HIV is still one of the most common causes of death among women in sub-Saharan Africa. It's still a very big issue. So it keeps engaging and it affects young people, which is also something that, you know. It's close to my heart.
Talare 1
It makes it easy to really, you really want to make sure that these people can have, that young people can live a full life and that children can be born free of HIV. Because AIDS is related to so many other issues. AIDS is related, as you said, to inequality between the sexes. It's related to social development. It's related to security. It has links with almost everything we do in life.
Talare 3
We heard a clip from the archive. This was an interview with Purnima Mane at UN AIDS from 2006.
Talare 2
One of the oldest documentaries in the archive is AIDS, Metaphor and Reality from 1986, documenting one of the first international AIDS conferences in Europe. The film not only collected some of the leading voices at the conference, but it's also one of the first films to let people with HIV to speak for themselves. A later film is The Longest Journey is the Journey Within from 2015, which shows how far we have come in treating HIV since the 80s. The film is a portrait of Swedish HIV activist Steve Sjöqvist. Anna-Mia, you were involved in this film. Can you tell us more about who Steve Sjöqvist is?
Talare 1
Yes, he's a fantastic person that I've met a number of times. And he was It's opened that he's a gay man and he was early on infected by HIV and also very close to dying from AIDS and one of the first people then who got the ARVs, the antiretroviral treatment medication in Sweden, which saved his life. And from then onwards, he's been an activist. His witnesses, his ability to communicate and his love in a very humane and warm-hearted manner, I think has made him an important focal person for the activism in Sweden and globally.
Talare 2
Yeah. And we're going to play a clip from this film as well.
Talare 3
For if we are truly created equal, then surely the love we commit to one another.
Talare 1
must be equal to all. Isn't that wonderful? Yes. As a 20-year-old walking on Christopher Street, it was like freedom.
Talare 3
It was like, I'm alive, I'm a person, acknowledge me. I don't have to hide anymore.
Talare 1
Well, I have exactly the same experience from 79 when I was walking here, and I thought it was, yeah. You're selling freedom.
Talare 3
We heard Steve Sjöqvist and Donald Johnson in a clip from The Longest Journey is the Journey Within. There are many interviews with activists in the archive. Steve Sjöqvist is only one of them. How important would you say activism is in HIV and AIDS history, Anna-Mia?
Talare 1
Incredibly important. It's thanks to the The activists that we are where we are today, actually, in terms of treatment, they were really pushing to make treatment available to everyone, free of charge, especially important to make sure that also the continent, which still has the highest burden of HIV, Africa, actually finally got access to treatment and has up until now, where we don't know what's going to happen with treatment, it's doing extremely well. adherence to the treatment is excellent. Treatment results are great. And that's, you know, thanks to sort of the unselfish work of many activists. That's also something that struck me when I went to look at some of the clips in the archive again recently, the fantastic engagement of activists across the board, both young people, but also that are themselves living with HIV, mothers. but also researchers, doctors, even Nobel Prize awardees and monks. Everybody was engaged into fighting this sort of this virus and the disease associated with it in the 1980s and in the 1990s. And people weren't quiet.
Talare 1
They really made their voices heard. And I don't see that today. That's really what struck me. It's quiet. And we know that silence kills.
Talare 2
And you think this activist involvement is quite unique for just the HIV response?
Talare 1
It is. It really was. Because I think it's so closely associated with life. And this was something that killed people in their 20s, 30s and 40s. Young people who hadn't even started life. Children were dying in hundreds of thousands every year. It touches people. It undermined societies. Parents disappeared. Orphans had to grow up without anyone between 30 and 60 being alive in some communities. Grandparents had to become parents again. Teachers disappeared. Nurses disappeared. Policemen disappeared. Everybody, sort of farmers, those who sort of built up societies, vanished. over a period of just a few years. This was something that had never ever happened before in the world and I really hope we don't have to go there again. But it was devastating, really devastating and something that we had never seen before.
Talare 2
Why do you think it's so quiet now?
Talare 1
I think that because there's so much else really occupying the sort of media space. We have the war. In the Ukraine now, everybody's talking about whatever the U.S. administration comes up with on a daily basis, like the tariffs or there's so many other things that are sort of occupying our minds and the media. And I also think that people sort of think that HIV doesn't exist anymore. We hear so little about it, actually. But many more people have died from AIDS than who were ever at risk of dying from COVID. For example, that was very, you know, a lot in the media, obviously, and scared a lot of people. And a lot of people are still infected and die on a daily basis. Even today, the incidence of HIV or the number of new cases, as well as the number of deaths due to AIDS, has more than halved in the last sort of decade, which is an absolutely amazing success. But still, more than 3,500 young people are infected every day. Most of them are teenagers even, or children, or really, you know, in their early 30s, 40s.
Talare 1
And every day also more than 1,700 people die from AIDS, a completely preventable cause of death. And that's tragic, but Now, as we see, you know, funding for drugs are being removed. These numbers could easily double. And the UN AIDS, the United Nations AIDS organizations, has predicted that unless, you know, HIV funding is restored in full, we will see 6.3 million deaths from AIDS by 2029, which is, of course, absolutely horrific. And we have, the treatment is there. And letting people die from a completely, and most of them very young, it's just horrible. And it needs to be brought to the attention of our politicians and policymakers. It's not only that we don't really hear much about HIV anymore, but another problem right now is self-censorship. And that so many clinics that are working with HIV and HIV treatment and prevention are really afraid of speaking out due to fear of having their funding removed. And people have lost their jobs, hundreds of thousands of people have lost their jobs in the past few months.
Talare 1
And they are really scared of speaking out. You can almost not find anybody who's worked with an HIV program in Africa who is ready to talk about the consequences of this. And because the American administration also removed all funding, sort of all development aid funding pretty much, in particular related to data collection, we won't even be able to document this and track the impact of the removal of funding. And not only the US administration is cutting down on development aid, also EU countries and Sweden is doing the same thing, unfortunately, when they should rather do the opposite, you know, and step up and try to fill some of this gap. And it's not much money. If you sum up all of the money that has been withdrawn from the U.S. administration, that went to PEPFAR, which is a fund, President Bush's emergency fund for AIDS research and treatment, and the Global Fund for money to the WHO that they've also withdrawn, and the USAID, it actually sums up to less than 1% of global GDP, you know, the gross development.
Talare 1
products. So it's nothing. It's a fraction of what we invest in defense, for example. So we can really easily afford both building up the defense, which is now the focus of all countries, it seems like, and still keep people alive. It's an obligation that we have. And it makes me really upset that we're not able to keep two important thoughts in our heads at the same time. And we can really do this. without sacrificing much in terms of, you know, funding that could have been used elsewhere. So I think that's really important to understand. It's not much money that's needed to save millions and millions of lives.
Talare 2
Yeah, and activism could play a part. And we have… Another clip from another activist. This is South African activist Mary Jane Matsolo. And she was interviewed in 2012 and explains why she is an HIV activist.
Talare 1
I'm an activist because I believe. I believe in human rights. I believe that people deserve an equal chance of life. I believe just because you have HIV, it doesn't necessarily make you any less different than that other person that is HIV negative. I believe that whether you are I have TB or you don't have TB. We both are equal. And this is why I became an activist.
Talare 3
The African countries are of special concern here. We have a few clips from the archive. We will first listen to two clips from 1998. Rose Bulia Kizito in the Rakai district in Uganda and Helen Jackson working in Zimbabwe.
Talare 1
We are in Rakai district. The epicenter of the epidemic, as they say. The first cases of AIDS were seen in this district as far as 1982. But by that time, not many people knew about the epidemic. And the first people to be hit were the business community. This was once a flourishing town, but right now there is hardly anything because of AIDS. We find that the young energetic people have already died. between the age group of 25 up to 40. They have died, leaving the old people. This district used to produce a lot of coffee and bananas for trade and was flourishing. But right now there is hardly anything because of AIDS.
Talare 4
Projections suggest that around one third of all children in the country will eventually be orphaned. Now potentially that's an enormously unstabling factor for the country as a whole. It's very serious in human terms, social terms for those children. And if they grow up poorly socialized, unable to go to school, without good care, without ability to develop self-esteem, skills and so on. That sort of number potentially means a massive destabilizing factor for society as a whole.
Talare 4
From an economic point of view only, all of the skill areas will become increasingly problematic with high labour turnover, increasing costs of training, recruitment and of course the loss of institutional memory and experience which you can't replace. The cost of benefits will go up, productivity will go down, the capacity to pay for the increased costs will go down. So while the needs are going up, the capacity to deal with them will be reduced. I don't think we have yet really grasped the full scale of what the long-term impacts are likely to be. There's no precedent in recent history of this type of incremental escalating death in the productive sector and among parents.
Talare 3
You've been to Rakai, Anna-Mia. How would you describe the situation today?
Talare 1
I was there about a month ago. And as mentioned in one of these clips, this is sort of the place where the first AIDS cases were reported in Africa in 1982. And Rakai is just by Victoria Lake. And it has a lot of mobility of fishermen and trucks and businessmen who are sort of handling the fish. It's also that mobility that made HIV spread along the transport routes and around Victoria Lake. And this is still a very high-risk area of HIV, even today. And when I was there a month ago, there was great, great fear because of the withdrawal of funding for HIV treatment. And I've been visiting a school every year since, over the last 10 years, and it's a school for HIV orphans. primarily, where most kids have lost one or two parents due to AIDS. And several of these children are also living with HIV themselves. And now their treatment is a threat. And when I was there, the treatment had already been cut. And parents had taken their children out of school waiting for them to die. So incredibly, incredibly sad.
Talare 1
Now they have found a few more sort of containers of drugs that will last them for another month or so. But there's great fear in Uganda that we will see the same horrible scenario again of child coffins along the roads rather than toys that we have seen the last 10 years. And that more children will be taken out of school, that more children will have to grow up without their parents. putting them at very high risk of dropping out of school or becoming infected with HIV themselves. So it's really tragic to see that we haven't come further and that these people once again have to fear for their lives.
Talare 2
And what can European countries and the European Union do now? You were talking about that before, but what can be done now?
Talare 1
A lot. As I mentioned, it's not much money. It's a fraction of what we invest into defense. And it's much less than 1% of that. So that's needed actually to save millions of lives. We have 20 million people on antiretroviral treatment in sub-Saharan Africa that are dependent on international funding. And of course, Governments in Africa are stepping up to try to cover some of the funding gap. But preventive activities like testing of young people so that they become aware of their HIV status and they can start medication so that they can't transmit the virus to their partners or babies has ceased. HIV testing is much, much reduced at community level. Condoms are no longer available. PrEP, as I mentioned, pre-exposure prophylaxis that can prevent someone at high risk of being infected with HIV has been removed to a large extent. There's still a priority of pregnant women living with HIV to make sure they are taking their drugs during pregnancy and breastfeeding to protect the baby. But since we're not testing any new people, so many more will be infected who aren't detected until they get sick.
Talare 1
And there's been estimates that only during this three-month of work stop, stop work, that has been announced by the US government when they have frozen all funding for HIV and development, 136,000 babies are at risk of becoming infected with HIV. We're going into a very likely scenario of having new HIV infections double. over the next few years. And as mentioned before, unless funding is restored in full, we will have 6.3 million deaths from AIDS over the next four years. And most of them will be very young people, young parents and children.
Talare 2
And how can we communicate this threat to the world?
Talare 1
Well, there I think actually, as a Swedish researcher, we have, or as people living in Europe, we have the freedom to speak still. We still have democracy. We still have a functioning legal system and freedom of speech, although we see that this is also sort of sometimes a threat and being discussed. We still have this opportunity to speak out, and we need to do so. Since our American colleagues have been silenced. And many of our African colleagues are also sort of self-censoring what's happening because they're so afraid of losing their jobs or, you know, the clinics being closed down. So I think we need to speak out. And the absurdity here is that there's also now a list of forbidden words, 250 forbidden words that the US administration has published. to prevent people from talking about these issues. So words like woman, sexuality, prejudice, HBDQ, contraception, even words like obesity, climate crisis, clean energy. I mean, most of the words that we use in research are no longer allowed because they are associated with the DEI.
Talare 1
diversity, equity, and inclusion concept that the U.S. actually introduced and sort of made everybody use who are involved in research on sexual reproductive health and rights and HIV. And now the same government is sort of making us not use these words. And all official documents that use these words in the U.S. have been removed and cleaned out from the websites. Databases are removed and even scientific articles. are now at risk of being erased from the public space, which is incredibly serious. This is a massive attack on academic freedom and on science as a whole, something we've never seen before. What's happening is really, really frightening, and we need to do something about it right now.
Talare 2
I think the library has downloaded or like… The backup of the PubMed service.
Talare 1
Yes, the PubMed is the database, the most important database for us as researchers for peer-reviewed scientific articles. This is where you can access all the results of quality reviewed research from the entire world. And this has also been financed to a large extent by the USA. And now, They are threatening to remove this funding, meaning that all research that has been conducted over the past decades is at risk of vanishing, being removed from the public space, which is something unthinkable for us in academia. I can't even imagine that anyone could go on and conduct research without having a resource like this. So I'm really grateful that the Karolinska Institutes Library is sort of safeguarding what's out there. But it's, of course, not enough. We need the EU to step in and take over, I think, the financing of this, to secure it. It's invaluable.
Talare 2
Thank you, Anna-Mia Ekström, for being with us today in this podcast. And thanks, everyone, for listening to us. This was the KB podcast, a podcast from Karolinska Institutet University Library. The publisher responsible is Miriam Nauri. And I'm Martin Andersson, librarian at KI.
Talare 3
And I'm Martin Kristensson, also librarian at KI.
Talare 1
This program is made possible by Beppo.
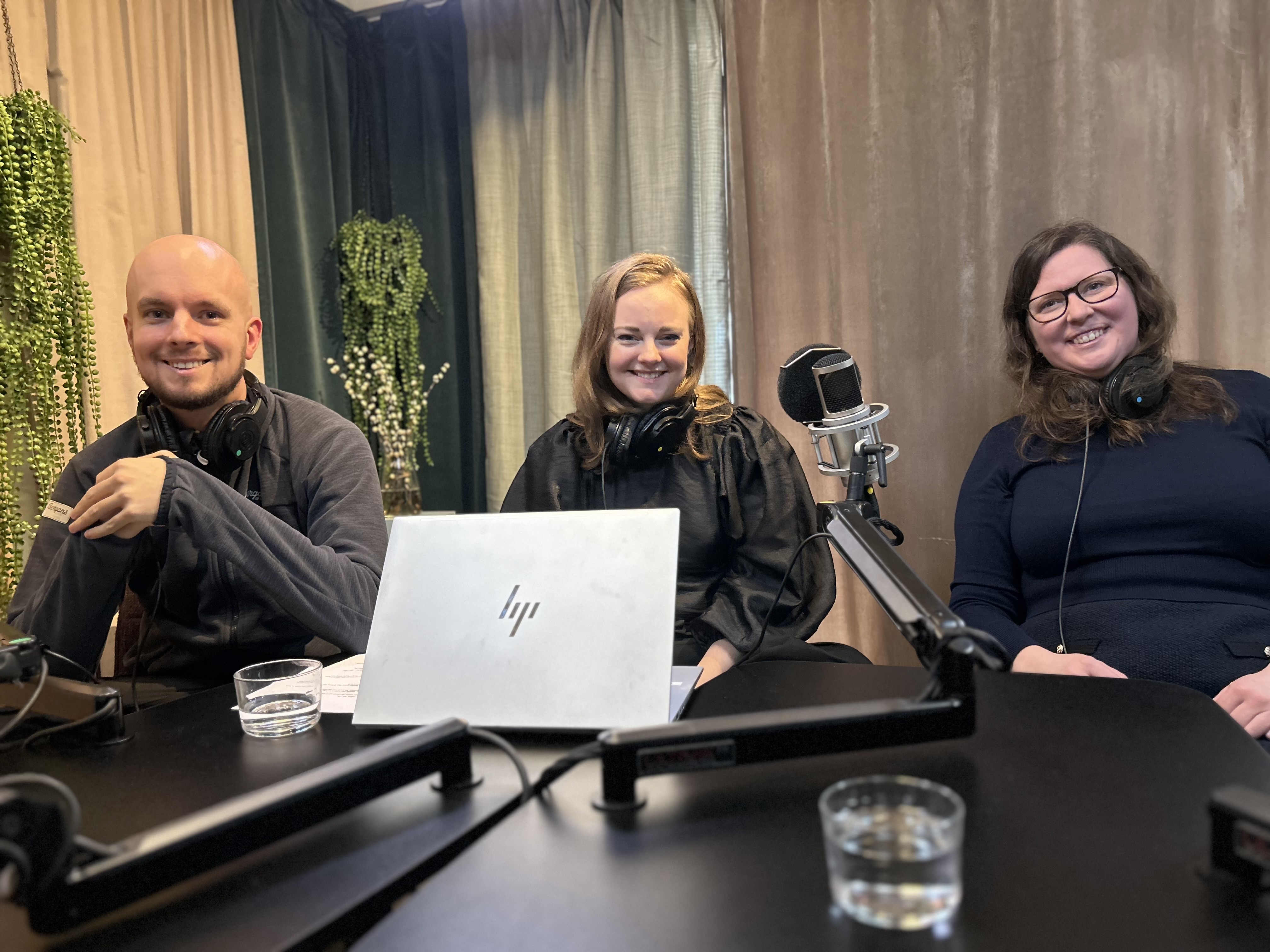
Episode 2: How are KI students using AI? The library discusses
In this episode of the KIB podcast we dig deeper into the academic perspective on AI: How do students use and reflect upon the use of AI in their studies – and how should they?
- How can I use AI to support me in my learning process?
- What’s happening to our brains? (Or to the intended learning outcome?)
- Is it cheating if I ask “my friend Bob” the AI to write my essay?
Related information
Transcript of the KIB podcast episode 2
This is a transcript of the KIB podcast episode 2. The transcript is made by AI (there might be minor mistakes).
Talare 3
Welcome
Talare 3
to the KIB podcast, a podcast by Karolinska Institutet University Library. I'm Lovisa Liljegrén, I'm a librarian at KI.
Talare 1
I'm Anna Borgström, a writing instructor.
Talare 2
And I'm Alvin Gavel, a bibliometric analyst.
Talare 1
So this is our second podcast on the topic of generative AI. And this time we have talked to a lot of our students and we have interviewed them about how they use generative AI in their studies.
Talare 3
Yeah, so in the last episode we talked about questions that we get at the library from our patrons, from our users. But in this episode we will focus on how students actually are using AI in their studies and how they are reflecting about their use. And the tools that they are using is mainly ChatGPT, not surprising.
Talare 1
Yeah, they almost laughed, some of them, that I asked because it was so self-evident to them that they would use ChatGPT.
Talare 3
But some other students also use Copilot, Perplexity, Notebook LM. I also heard one student using BARD. So we see that they are using different tools, but ChatGPT is probably the most dominant tool.
Talare 1
Yeah, I think Gemini was mentioned as well. And the thing is they're using these tools for all kinds of things, aren't they?
Talare 3
Yeah, for searching information, writing, reading, coding.
Talare 1
And I mean they also have some concerns and interesting reflections. So what we're going to do today is we're going to dig deeper into all these ways that these students are using AI and the ways they're thinking about AI use.
Talare 3
But first we're going to start with the students that are not using AI. Because there are some students that are not using AI and it's not only because they don't know how to use them and so on. Some of them have decided not to use the tools, right?
Talare 1
Yeah, I think, I mean there are some who are still uncertain about the tools and they don't quite dare use the tools because they don't know what's right. But there are some that definitely know about the tools but they don't want to use them.
Talare 3
Yeah, and the reason behind why they don't want to use these tools can be different. There can be ethical reasons, for example, environmental reasons.
Talare 2
Yeah, these models use a lot of power to first to train them in the first place and then to run them as well.
Talare 3
Yeah, exactly.
Talare 1
Yeah, and you need a lot of water and you need minerals that need to be mined, all kinds of things. But then also another ethical concern is that all these big companies are really profiting of the hard work of a lot of individuals, smaller firms that are getting no credit for all this hard work. So that's interesting. And I think another way that, another reason they don't really like using these tools would be that, I mean, they don't trust the tools. I mean, either they've heard about these tools not being so great or they have used them and they weren't quite happy with their performance. What do you think? Is there a sense of pride as well?
Talare 3
Maybe, I think that Alvin has lots of things to say here.
Talare 2
Yeah, I'd start by saying that they're basically correct in not trusting them. They aren't trustworthy, like it's been tested several times now. They very often output things that aren't true, which we discussed last time. People talk about hallucinations, and I think it's important to realize that there isn't actually a distinct error state of these language models that is a hallucination. What they're doing all the time is just generating text that statistically speaking sounds kind of plausible and that's all they do. Like they're not truth producing engines, they're just plausibility producing engines.
Talare 1
Yeah, and I think some of the students have heard about that or they've experienced it and they don't want to use those tools.
Talare 3
Do you think that these students who decide to not use the tools, do you think they will kind of like get behind in a certain way? Or do you think they will be just fine like without using them?
Talare 1
I think this is difficult. I'm not sure because I've heard researchers say as well that if we're not using these tools, we're going to fall behind very quickly. So I'm not sure about it, but I don't feel so concerned about those students because they have made a very conscious choice. Some of the students I'm thinking about though are the students who don't really dare using the tools because they don't know exactly how they're used or a teacher told them that using these tools is cheating or using these tools is bad. So they, and still apparently some teachers say that, Well, you can use them, but don't use them for this. That's cheating. Don't use them for that. We're gonna dig deeper into that. But they're also uncertain about how to use the tools. And I think those students, they may risk falling behind if they just don't know how to use the tools, because I think they're not trustworthy for some things, but I think at least several students have found a good use for them otherwise.
Talare 1
But what did the students say?
Talare 3
Well, I talked to lots of students that said that they are using the tools to find something real quick. They want to, when they've been to a, they've heard their teacher talk about something and they want to just get that confirmed or they want a clarification of something that the teacher said or something like that. That's how I've heard some students are using these tools.
Talare 1
I think a lot of them are actually using these tools to search for information. And one student, I think this was funny, one student said that Google is actually disastrous compared to ChatGPT. I get so much better answers from ChatGPT. I was a little bit puzzled, but the student also said, well, I may have to whip ChatGPT a bit to get the answers I want, which I came to understand that she was prompting the model in a different way.
Talare 2
Yeah, I think one of the reasons for this is simply that Google has gotten worse. Like I think we've all noticed that the search results are worse than they were five years ago. And there are reasons for that. Essentially, they want to increase engagement, which means that if you get the result you wanted quickly, well, by definition, you have very little engagement because you just go to the thing you wanted. Whereas if you spend a lot of time going, where is it? Where is it? Well, you're more engaged. And this is a problem through lots of different products right now. Facebook has a similar problem, but that's not a topic for today.
Talare 1
No, but I mean, at least some students relied on these tools. I mean, they also knew that sometimes the answers weren't accurate and they experienced that.
Talare 3
Yeah, that was really interesting that they were all reflecting on that. They were all, or at least all the students that I talked to, they were reflecting on that they knew that the tools sometimes did give wrong answers. And like they used background knowledge to see if the answer they got was right or not. Or they sometimes looked up the answers. Like, for example, I had one student who said that they did go to like the teacher's PowerPoint to see if the answer was correct, that they had gotten from ChatGPT or not.
Talare 1
Yeah, so that's wise.
Talare 3
Yeah, and I've also heard students say that they have realized that these tools, for example, they cannot count. So they realized areas where they cannot use these tools as well. But they have realized this through trial and error. It's not like they have read about it, is my impression.
Talare 1
I think so too. I think perhaps both. They've heard about it, but then these tools were so good when they used them, but they have also seen the other sides. So they seem to have a fairly nuanced view in that respect. But some of them also noticed a bias.
Talare 2
So what kind of bias are we talking about here? Because there are many kinds in this specific field.
Talare 1
Yeah, I think they were thinking about a political bias. What do you have to say about that, Alvin?
Talare 2
Well, this is a bit complicated because the people behind the language models like ChatGPT and so on, they try to tweak the models to simply not take political positions. For obvious reasons, like there's nothing to be gained from being known for having a product that took political positions, so and so. So nowadays, they basically just be like, nah, if you ask it something political. But inherently, what the model would otherwise do is simply to reflect the training data. That's how all language models work. They just reflect the statistical properties of their training data. Which is, of course, why they tried to make them not take any political position, because you don't really want your language mode to suddenly express the political views of your average 4chan member, for example. But the thing here is, of course, that what is a political position is itself a political position. We played around a bit earlier with the new light model DeepSeek that was being made in the People's Republic of China.
Talare 2
And overall, it's pretty good, but it has a few quirks. Like it can explain to you how, of course, Taiwan is inherently a part of the rest of China. There isn't actually a Republic of China separately over there. And it can also, if you ask it about did anything interesting ever happen on Tiananmen Square, it will explain that no, no, nothing ever happened. Unless you forget how Tiananmen is spelled and spell it wrong, in which case it might tell you that yeah, a bunch of people died there during a massacre.
Talare 1
Oh wow.
Talare 3
Yeah, this is interesting. And you did, to sk the same question to Chatjiputi and to Deep Sik, didn't you?
Talare 1
I did. I talked about the Great Wall of China. And I mean, some things were very similar, but what stood out to me, I mean, I wouldn't perhaps have thought so much about it if this wasn't a test, but what stood out to me was that, especially towards the end, the Deep Seek version emphasized not just how this had enormous historical value and cultural value, but also how the wall itself was a testament to the hard work of protecting a nation. That was not the answer I got from ChatGPT. Of course, most of the answers were similar. So I mean, I'm not surprised that students would notice this as well. I'm impressed with how much our students really reflect on these things and how much they have realized.
Talare 3
I have a question from a student here that I would like to ask maybe you, Alvin, and see if you can answer it. Why does it seem to always find solutions to problems, even if the solutions aren't realistic applicable? It seems overly positive. Yeah.
Talare 2
So this is also, well, I think there are two things here. First of all, they're models of what language tends statistically to look like. And most written texts of a person being asked for a solution tend to contain somebody proposing a solution. It's fairly rare that you read a text where somebody asks about something and just get answered, I don't know, or sorry, this is unsolvable, even though it happens. So it's sort of just by knowing statistically what the text tends to look like, it knows that there should be something that looks like an answer here. In addition, they tweaked the models to be very, very friendly and helpful. Again, because they don't want to reflect this, how people statistically tend to talk to each other on the internet. And well, you have a model that tries to be friendly and helpful and is trying to give you something that plausibly looks like a solution to a problem. And it doesn't understand the problem because why would it? It's a language model, it only understands language.
Talare 2
So it will output something that is solution to your problem shaped. Which will of course won't actually be a real solution to the problem because it has no real ability to do that kind of thing.
Talare 3
I do also find it interesting that the students are using large language models to fact check things or to find facts fast.
Talare 1
Yeah, I mean, one student even called it a quick Wikipedia. Didn't another student say that they fact checked the information from ChatGPT with information from Gemini?
Talare 3
Yeah, I had a student who said if they are not happy with the answer that they get from ChatGPT, then they go to Gemini.
Talare 1
Oh, okay.
Talare 3
And what I also found interesting is that many students said that it's when they feel that answer is not correct, when they feel that this might not be as it should be, then they go further and check the answers. So it's about a feeling that it's not correct.
Talare 1
And I guess that gut feeling is based on critical thinking.
Talare 2
I would like to talk about AI summaries.
Talare 1
That's what I want to talk about too.
Talare 3
Yes, and that is something that the students said that they are using these tools for, to get summaries, but also to summarize their own texts.
Talare 1
And I think some of them translated, summarized, and simplified the scientific articles with these tools. And yeah, I mean, I totally get it. And I think that's where one student actually said something that I thought was so insightful and still quite funny. You kind of know that you don't want to over-rely on these tools. And the student also said that teachers have kind of said that you shouldn't use them too much. But what she said was that every day I think that today I'm not going to use ChatGPT. But then I end up using it anyway because it's so good. And she did reflect, I mean, am I getting lazy when I do this? Alvin, you have something to say about this, I think.
Talare 2
I may have a lot to say. I've honestly been a bit obsessed with the topic of AI summaries recently. Because I keep hearing people tell me this, that they say, oh, I asked ChatGPT for a summary of this thing and it was really good. Or, I thought this paper was a bit hard to understand, so I asked ChatGPT for a simplified explanation and it was really pedagogical. The thing is, I tried doing this, taking reasonably complex texts that I understand well, where I know what they're really about, asking language models to summarize them. And what comes out is plausible looking nonsense. So I wonder, am I doing something wrong? Is it that there's something in the prompting web that I'm doing badly? But several people, or not just people, there have been large scale studies on this. Like BBC, they tested whether AI could summarize the news. It cannot. It ended up creating pure nonsense a lot of the time. The Australian Securities and Investments Commission checked if language models could summarize their reports.
Talare 2
They can't. They again make things that look kind of plausible unless you actually understand what the original text is about. So that's the thing. When you're asking language models for summaries, you are going to get something that sounds plausible and maybe feels really pedagogical and well explained. Because that's kind of what they do. But they don't understand the original materials, so they are about as likely as not to be giving you plausible nonsense.
Talare 3
One question on this topic as well is that I've heard students who are using large language models, they use Notebook LM and they uploaded a book, like a course book there. And then they started to chat with the book. So it was kind of like another way of reading the course literature. What do you think about that, Alvin?
Talare 2
It seems like a different version of the same thing. I mean, you're not actually chatting with the book, you're chatting with a language model that has some pieces of the book in its context window. It still isn't suddenly going to understand that book. So it's still probably going to tell you things that sound kind of correct, that make you go, yeah, that makes sense, that makes sense. But if the person who wrote the book hears it, that person will probably have a heart attack or something because it will be horribly wrong in many places.
Talare 1
One thing that I found interesting was that, well, when we talk to students and they said, well, I mean, I use these tools to summarize texts and I do that all the time. And I have to admit, initially I felt like, hmm, you know, that sounds kind of not so great. But then I realized with almost all of them that they did always have this sense, is this correct? Because I do know a lot about the topic. Or does it seem to make sense? So initially it sounded like they just did this, but then it turned out that they didn't. I mean, they did use the tools in a much wiser way than I initially.
Talare 3
They are always thinking and reflecting on their use and about the output that they get and so on. So we do have great students at KI.
Talare 1
Yeah, no we do. But I still think that some of them were concerned that, well, maybe I'm not getting as much practice reading scientific articles as I should. And I think maybe that's potentially a concern, but I do think that they're not as uncritical as people may think when they hear that they're using these tools to, yeah.
Talare 2
I think you're correct. I just think being critical is of much less value than people tend to think it is. I'm going to share an observation here that I heard from a physicist, that a physicist who work with radioactive substances will quite often carry them around in just their shirt pockets. And if you ask them, hey, should you really be carrying a radioactive substance next to your chest? Is this really a good idea? They're like, yeah, but I'm a nuclear physicist, I'm aware of the risks. And somehow they assume that just being aware of the risk somehow makes the actual risk go away. So if you're using very unreliable tools to do study and to do research, you can be critical about it and reflect about it, but I'm not sure that's going to have much effect in the end to the actual learning process.
Talare 3
Yeah, but this is also like something that we did talk about a bit last episode, that it's getting harder to actually critically evaluate the things that you get out from these tools and it's getting harder to to stay critical because it's the technology is so opaque and it's so it's quite hard to to. But still I did get happy that they do reflect and like even if that won't be enough in the it's still something.
Talare 1
Yeah, no, I think so too. Another thing I wanted to say about this was, I mean, of course many students do use these tools to polish their texts, polish their writing. Many of them seem quite content with what they get. Still there are, I mean, there are a lot of questions like how much can I outsource to these models? How much has to be my own? And of course that's also linked to critical thinking and not just critical thinking but this constant awareness that, okay, what am I really learning here? But also that what's okay that wasn't completely clear.
Talare 3
Yeah, but I also had a student who said that they don't feel confident using ChatGPT for example for a thesis because they said that then I have to be responsible for everything and I have to know my material so well. So then it's quite useless to use these tools. And I found that quote to be very interesting and also that they are very much reflecting on the use.
Talare 1
I mean, it does make me a little bit curious about how you use these tools because I mean, clearly if you ever use these tools, you still have to be responsible for everything in the text. You have to be accountable. So, and it's still possible to use these tools, but perhaps not in the way this student.
Talare 3
Yeah, and I must say that they did add also that they sometimes use the tools to translate text or something like that even in the thesis. But I found that quote to be very interesting. Yeah, yeah.
Talare 1
No, I think so too.
Talare 2
Yeah, I'm just going to prove that I'm not entirely opposed to AI by saying that, yeah, translation, that's a pretty good use case for them. They're good at that.
Talare 1
But you should still double check. I mean, one thing we have noticed is that some of our students use ChatGPT or other AI tools to code.
Talare 2
Yeah, I do too. I don't use ChatGPT because it's not good at it. I use a thing called GitHub Copilot. Essentially, what it works is you're writing your code and at some point it will figure out the pattern in what you're doing and suggest that it looks like you're doing this. Do you want me to fill this in for you? And you can press tab and then you got the stuff. And that's the kind of language model I really like because inside, all language models do that. They're all a type of auto-complete. ChatGPT is, for example, Gemini is. But the auto-complete part is hidden away inside of the chat-shaped interface and that makes it much harder for you to see what's actually happening when you're using them. This one is much more transparent about what it actually is that it's doing. And that way it ends up being pretty useful. It's not magic, but I code a bit faster than I would have otherwise.
Talare 1
And I think at least from what I've understood that it's mainly our advanced students, our master students who are using these tools to code. And they seem quite happy because they're saving time, but I'm happy to say that they are also critical of the output. But they have been asking questions also like, well, what am I really learning? Should I be learning this? Do I need to know all of this? And I think it is important to understand the coding process in order to use these tools, right?
Talare 2
Yeah, I'm going to say, you shouldn't. If you're learning to code, you shouldn't use a tool to help you. You should just do it. But once you know how to code, then it becomes really helpful. But first of all, it makes you do routine things faster. But also every once in a while, you'll be trying to solve a problem. It will suggest something that's completely different from what you would have done. And you'll sit and be like, hold on, why did it do it that way? And sometimes it's just wrong, that happens. But some of the time you actually learn something that you didn't know about the language you're working in. And that actually helps the learning process.
Talare 1
Yeah, speaking of the learning process, I think quite a few students use these tools.
Talare 3
Yeah, what I found interesting is that lots of students are using the tools to. They are uploading old exams and also like the intended learning outcomes from their courses and then they ask the models to create study quizzes that they are using to learn something for their exams and so on. And I did find it quite surprising that lots of. Actually quite a few students are using it this way to actually increase their own learning as a learning tool.
Talare 1
And I think some of them also thought about not just how can I use these tools to help me but how can I integrate the tools into the learning process? And I was particularly happy with that question or those kinds of questions like acknowledging that it's not just about doing things more quickly or just writing a better text but it's also about learning.
Talare 3
Yeah, and I did find it interesting too that most of the students did reflect upon their own learning and like what am I learning when I'm using these tools and how can I use them as a learning tool instead of like just doing something real quick and.
Talare 1
Yeah, and I mean some of them were concerned about that. One student said well these tools are clearly great but what do we learn? What's happening to our brains? So I mean some of them are aware and they're adjusting the way they study to that but some of them did seem to think that maybe I'm not learning as much as I could have. Maybe I'm getting a little bit lazy with this.
Talare 3
Yeah, I had another student who said quite a similar thing. She does use the tool and she like we're all busy people like we all have lots of things to do and when you're a student you're probably torn between different things and you have lots of things to do at the same time. She thought that the tools they do limit her learning but that she still uses them because her time is so constrained like she.
Talare 1
And I'm thinking like this is also bordering on another topic which is I mean how can I use it in a way that's okay? And now we're talking more about okay so that I learn but also okay so that it's not cheating. And one way of phrasing this that I thought was really nice was well how can I use it in a way that actually supports my learning and not as an ugly shortcut? And I think that kind of encompassed both of those aspects. And I mean what's your impression? Do students always know what they can do and can they talk to their teachers?
Talare 3
I think sometimes they can talk to their teachers but it's sometimes it's quite unclear but it's also unclear for the teachers how to deal with the question. So of course sometimes it can be more uncertain like how they are supposed to use the tools and how they can use the tools and so on. So of course that is.
Talare 1
Yeah I think no one said that they couldn't talk to their teachers but some students said that well we can talk to our teachers but I mean they don't know everything about these tools and they don't know exactly. Of course the teachers try to be as clear as possible but another thing that I thought was quite clear at least with some students, well even if there are some kind of guidelines or things to consider when using generative AI, it wasn't always super easy to take those guidelines and apply them on their specific situations.
Talare 2
Yeah, I'm gonna say I think that's a more widespread problem than just in studying. That in many cases the legal situation is unclear. Like to what extent do you have intellectual property rights to things that have involved generative AI at some step? It's a bit unclear. It will probably differ from one country to the next because there are things that sort of where reasonable people can differ about how you should interpret the concept of copyright and intellectual property in general.
Talare 1
Yeah, there were quite a few questions and you could see that students really did want to be able to ask and some students have come up to me with very specific questions. Can I allow an AI to write an entire text and then work on it thoroughly? My answer had to be yeah, I don't think so. It doesn't sound like you're perhaps doing enough to fulfill the intended learning outcomes.
Talare 3
Yeah, because that has to always be the leading thing like how to. You need to think that you need to learn something, especially as a student. That is the goal of like why you're studying at all. Like so of course that is the most important.
Talare 2
Yeah. I'd also make a call back to our friend Bob from the previous episode that in general if you're wondering if something is okay to do with an AI, replace it in your head with my friend Bob. If I say I had my friend Bob write an essay for me and then I just worked on that essay and now I kind of feel like it's my own essay. I'm probably cheating by the rules of the school if I do that.
Talare 1
One thing that students also said was that either they said it out loud or I kind of understood it from our conversation that if teachers ever say using AI is bad or using AI may be cheating and leaving it at that, the students felt so insecure about talking to their teachers. They were kind of feeling like they were doing something that wasn't allowed. Even though some of them were using things that other students did and felt confident about because their teachers may not have said those things.
Talare 3
Yeah, so it might be great for teachers to actually talk to the students about AI. And the students that I talked to, most of them said that their teachers did talk about AI in one way or another, even though they thought that they could talk more about it or they could be more clear about how they can actually use AI in their studies.
Talare 1
I mean, we're still in a super early stage. Perhaps some of the first impressions to AI were like, oh, it's cheating. And I mean, that was only like a little bit over two years ago.
Talare 3
Yeah, and then I find it so interesting that we can see that the students, most of them are thinking about how they can use the tools to actually increase learning. Most students don't want to cheat. Alvin?
Talare 2
Yeah, there's also a bit of an elephant in the room here that I was thinking about, should I bring this up or shouldn't I? Because many schools and universities and so on have rules saying you're not allowed to use generative AI. Many use AI detectors that are supposed to check through a text and identify that it looks like it's written by a language model. They don't work. None of them do. So we're in a kind of uncomfortable situation where there are rules that are technically impossible to enforce.
Talare 1
Luckily, I don't think I know about any such guidelines at KI. No. But you're completely right. And I mean, some of the students even said that. I mean, it's impossible to focus on AI detection.
Talare 2
Well, at the end of the day, we don't give legal advice. We have been asked about this, but we can't tell anyone what is strictly speaking legal and what isn't in terms of intellectual property around AI.
Talare 3
Because what I found so interesting also talking to students is that they know most of them are using the tools. Most of them know how to use them. They have used a trial and error approach and learned how to use them, learned what works and what doesn't work. But when I started asking question about like, so what is it? What is AI? What is a large language models? They often didn't really know the technology behind.
Talare 1
Yeah, that was my impression too, that they hadn't quite thought about how these models work. But also I think sometimes they, some of them said that, well, we don't need to know anything else. We know how to use them. But then when we asked a little bit more, it turned out that there were a lot of things that they didn't know. And they were actually quite aware of that, that they didn't know. So I think there's a gap somewhere here between what students think they know if they don't really reflect on the topic and what they actually know.
Talare 3
Yeah, I agree.
Talare 2
Yeah, this is the thing I worry about a bit because of course your students are kind of correct in that we use technology that we don't understand all of the time. Like we have a laptop here. I mean, I'm even a programmer, but most of what actually goes on inside there, I have a very vague idea of how it works. But when it comes specifically to language models, it's much harder to realize that you don't know what's going on there because they're sort of, they're imitating the behavior of a person, right? They're acting like they're chatting with you, which strictly speaking they're not. They're doing internally a kind of auto-complete. They don't even know what parts you have written, what they have written inside of the conversation inherently. But because of this human-shaped interface, you kind of just fall back on your intuitive understanding of how human beings function. And that sort of shapes your expectations on what the language model can do. And that means people tend to get really, really blindsided when it suddenly does something weird.
Talare 2
Like saying something false or as happens occasionally, outright threatening you out of nowhere. There was a famous case with Gemini did that, which you may have heard of.
Talare 3
Yeah, it is a question like how much do you need to know about the technology behind to also stay critical? Like how much do you need to know about large language models to use them in a good way?
Talare 1
But I do think that even though we saw that there is this gap between what we think we know if we don't think about it and what we do know, we did see that students are using generative AI to a much greater extent than a year ago. And also in more sophisticated ways.
Talare 3
They want to learn.
Talare 1
Yeah, and that's in line with a very, very recent Nature article about students use of generative AI. That they're finding these really sophisticated ways and at least many of them are aware of the pitfalls.
Talare 1
Some of the students had some more philosophical questions about generative AI.
Talare 3
Yeah, so this can be our final question here. Is it possible for AI to gain enough intelligence to start coding itself to an infinitely large intelligence? What would be possible scenarios if this was to happen?
Talare 2
Okay, I'll try to take this one. If you're listening, Måns, this one is for you. So, the very short answer to the question is that in principle, yes. But with the technologies that are being marketed as AI right now, no, don't worry about it. Don't lie awake at night being concerned about this because it's not anywhere on the near horizon. But a slightly longer answer is that, I mean, all of our AI tools are tools. They're made for some specific purpose, like classifiers. Look at the images and say, that's a cat, that's not a cat, that's a thing. Language models, all they're doing is fundamentally to auto-complete a text. But there is this dream that's been around for a very long time about something called artificial general intelligence. An AI that just is more or less as intelligent as you or more. So they can handle general tasks as well as you can. Like, you know, the classical robot that you tell it to drive a car and just sits down and drives a car, you tell it to write poetry. And in most stores it will fail because they're trying to make some point about the limitations of machines.
Talare 2
But you can imagine the AI that also actually writes poetry, right? But that has led to worries about something called the alignment problem. And discussions about that tend to get a bit more technical than they need to be. You can talk about reward functions and so on. But you can understand it fairly simply like this, that if you have this kind of general AI, effectively you have a person, right? It has a picture of the world, it has goals it wants to achieve, right? But here's the thing, different people have different goals. Kind of crazy, but we do. And if any of us right now suddenly got like dictatorial power, just reshape society as we wanted, any of us would do something that the rest would consider to be absolutely horrible. We'd go like, why are you doing this? You're doing horrible things. And we handle that by not giving an individual person dictatorial powers. That's the only solution we've ever come up with. And it turns out that's a bit hard. Sometimes you discover that, whoops, that guy got dictatorial powers anyway.
Talare 2
And now he invaded Ukraine and we don't even know why. That sort of thing happens at a fairly regular basis. So the question is, if you have this super, this AI which is as intelligent as you or more, is it possible it will suddenly figure out some way to take power and use that to do something that from your point of view as a human being would be horrible? And then there's the second question here, what if this AI, what if it's just, you try to prevent that by making sure your AI is just a little bit smarter than you but not more, so you can see if it's suddenly trying to get hold on power, you can tell it knock that off or I'm shutting you down, right? But let's say now it knows how to program and it can access its own source code, so it starts making itself a little bit smarter. So you have this AI that's kind of bright today and then it's really smart tomorrow and then it's super smart two days from now and then suddenly you have this thing that you can't predict what it will do and suddenly does some things that you realize too late that whoops it just took over the entire world and now it's Skynet and it's doing something terrible.
Talare 2
And that whole scenario, it could in principle happen, but not with the technology that we're working with today. ChatGPT is not going to turn into Skynet and kill us all. So you can all relax. I hope you're glad for your luck.
Talare 1
I hope you ended on that note.
Talare 3
Okay, but then I think we're done. Yeah. Yeah. So this was the KIB podcast, a podcast from the Karolinska Institute at University Library. The publisher responsible is Miriam Nauri and I'm Lovisa Liljegren, a librarian at KI.
Talare 1
I'm Anna Borgström, a writing instructor.
Talare 2
And I'm Albin Gavel, a bibliometric analyst.
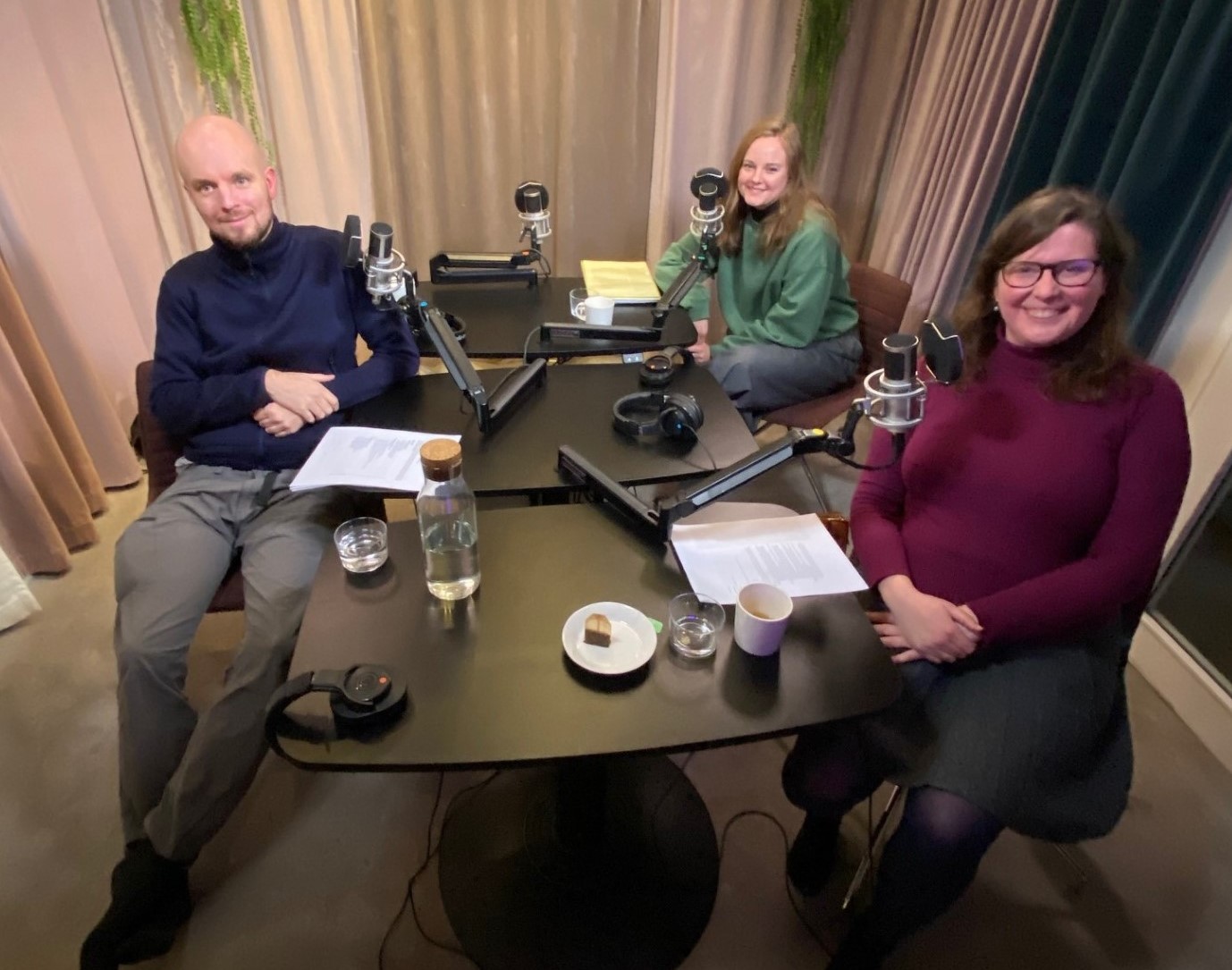
Episode 1: Your questions – our answers. Library people talk about AI
In this episode of the KIB podcast Alvin, bibliometric analyst, Lovisa, librarian, and Anna, writing instructor, discuss questions from colleagues and students at KI on LLMs (large language models).
- Does AI think?
- Does AI know everything?
- Can I trust "my friend Bob" the AI?
Related information
Transcript of the KIB podcast episode 1
This is a transcript of the KIB podcast episode 1. The transcript is made by AI (there might be minor mistakes).
ALVIN
Welcome to the KIB podcast, the podcast of the Karolinska Institutet Library. I'm Alvin Gavel, Bibliometric Analyst.
LOVISA
I'm Lovisa Liljegren, Librarian.
ANNA
And I'm Anna Borgström, Writing Instructor.
ALVIN
This episode is going to be about large language models. Most of it is going to be a discussion where we try to answer questions that the library has received repeatedly from people at Karolinska Institutet. So we have a little stack of questions here that people keep sending to us every once in a while.
LOVISA
Yes, but I think that maybe we can start with, I found this tool called Notebook LM from Google that creates something that sounds like a podcast. You can give it papers, you can give it web pages and so on. So I gave it the staff pages about us. So I gave it a staff page on you, Anna. I gave it a staff page on you, Alvin, and on me. And I think we can listen to the output and then we can talk a little bit about that.
NB1
You mentioned we have profiles of three KIB staff members.
NB2
Yes, we do. Let's start with Alvin Gavel, a Bibliometric Analyst with a background that's, well, frankly, out of this world.
NB1
Oh, tell me more.
NB2
His research background is in astronomy, astrophysics, and cosmology pedagogy.
NB1
Wow.
NB2
It seems his interests lie among the stars. He's published work on topics like the history of astronomy education and even the use of virtual reality in teaching astronomy.
NB1
That's amazing. I'd love to pick his brain about how his experience in those fields informs his work with bibliometrics at KIB. Right. It must give him a unique perspective on the evaluation of knowledge and the impact of scientific discovery.
NB2
I would imagine. So now let's move on to Lovisa Liljegren, a librarian.
NB1
Okay.
NB2
Who plays a key role in teaching information searching and reference management. Skills that are absolutely essential for any researcher, especially in the digital age.
NB1
Especially with the sheer volume of research being published these days.
NB2
Right. It must be like trying to drink from a firehose.
NB1
It really is.
NB2
What's particularly interesting about Lovisa is her special interest in AI and information literacy.
NB1
That's incredibly timely. With AI tools like ChatGPT becoming increasingly prevalent, it's more important than ever for students and researchers to understand how to use them effectively and responsibly.
NB2
Right. It's not just about using the tools themselves. It's about critically evaluating the information they generate. And understanding their limitations. Lovisa is clearly at the forefront of this emerging field.
NB1
And she's based in Flemmingsberg, but also available in Solna and via Zoom.
NB2
Yeah. Talk about meeting people where they are. And the last person we have a profile on is Anna Borgström, a writing instructor. Anna is clearly passionate about helping students develop their academic writing skills. She teaches group workshops, offers individual consultations, and has even presented at conferences on the topic. But what really struck me was her recent focus on teaching students how to use sources effectively, avoid plagiarism, and navigate the ethical considerations of using AI in writing.
NB1
That's really fascinating. It speaks to KIB's commitment to not just teaching practical skills.
LOVISA
Okay. So this was Google's Notebook LM.
ANNA
Wow. That's interesting. I didn't know all this about you, Alvin.
ALVIN
Well, this is where it gets weird because that was sort of half true and half insane lies. Because I have worked in astronomy and teaching. I haven't really mixed those. I don't recall teaching the history of astronomy education ever. I also haven't done anything with VR in astronomy teaching. I've worn a VR headset for a little while one time. I hated it because I got seasick. That's kind of my entire contact with the VR field. So what we had there is a pretty clear case of what people tend to call hallucinations in generative AI. I don't really like that term because it's not actually, you know, qualitatively different from what the model does at any other time. And I know you, Anna, also don't like it for several reasons.
ANNA
I don't like it because I think it sounds as though we think these machines have consciousness and that they're doing something or that they have a purpose of doing something. And I think that gives us a weird sense about them. Alvin, would you like to explain a little bit more about large language models?
ALVIN
I mean, you can get really far just by understanding that they are literally what the name says. A large language model is a large model of language. Like they're purely statistical models of what human language tends to look like. And with this particular architecture that's behind, for example ChatGPT, they essentially try to predict, given a string of words, what should the next word be? I'm simplifying a little bit here. Some of you listening might be yelling that, no, no, no, you don't mean words, you mean tokens, but whatever. This gives you the basic idea of what it does. And it so happens that if you have a really, really good statistical model of language, you have a model that will output language that happens to most of the time be true statements. Like it's more likely to output the sky is blue than the sky is green. Or fire is hot is more likely than fire is cold. That's kind of as far as it goes. Like it doesn't know what the color blue is, or what hot is. It only knows that these words tend to go together in a particular way.
ANNA
Yeah, but then I mean our presentations, they were a little bit funny, right? And super positive of course, and that's what you often get from these tools. But ours were a little bit more true, so to speak.
LOVISA
Yeah, and we need to know that the data that we gave this is that on our staff pages, Anna, we have written more about ourselves than Alvin has. On Alvin's staff page, you can find articles that he has published, but you haven't written a text about you, which we have done. So of course, there is more data from us and less to make things up from.
ALVIN
Yeah, which meant that it, well, I would say that interpolated, I prefer that to saying hallucinated. It's just given what had been put into the context that I had been working with astronomy and teaching, sentences like he has been working in education of astronomy throughout history and so on, those are plausible sentences. They don't surprise you that they're there unless you're me and go, what? When did I do that? Obviously, then you'll be a bit confused. And that tends to be the case most of the time when you'd say that a language model hallucinates, that it says something that's, yeah, I mean, sounds reasonable and sounding reasonable is kind of all that actually encoded in the statistical model.
ANNA
Yeah, and I tend to think about these models as a little bit like the kids pastime of connecting dots. You know, when you have dots with numbers and you're supposed to connect every dot and you get a picture. And that picture is, I mean, it often looks like something. It doesn't look exactly like the animal that you're supposed to, what the animal actually looks like, but it looks okay. But then I think if those dots are a little bit more sparse, you may miss something like a tail or an ear. And I think, is it true perhaps that since we had less information on you Alvin, to give to the large language model, that it kind of tried to connect some dots in a statistically likely manner, but then made some connections that were in fact not the same as the true situation.
ALVIN
Yeah, I suppose. Yeah, you could look at it that way. And of course, always keeping in mind that it never ever tries to say true things. It only tries to make, you know, statistically plausible sentences.
ANNA
Yes, exactly. And it doesn't have a mind of its own. It just does what it's programmed to do.
LOVISA
And I think maybe we can start with the first question. And that is about getting feedback from a large language model. So I can read the question maybe.
ANNA
Yeah, go ahead.
LOVISA
So I wrote an introduction to my text and I asked an LLM to improve the text and it got so much better. Can I use the LLM's version instead?
ANNA
Well, I'm happy you found the large language model useful, I would say. But I would say you have to be super cautious about getting feedback from these tools. And one reason is that, well, you will often get suggestions or rather rewrites of the text. And these rewrites, they're not based on, you know, rules about language or rules about or facts about the content, but they're just based on statistical significance. So that means, for example, if you've written treatment effectiveness and you get a rewritten text with treatment efficacy, these two are very much different in a medical context. However, these large language tools, they've been trained more on everyday language and there is a difference between effectiveness and efficacy. But the difference is not as pronounced as it is in a medical context. So, well, you can get feedback and sometimes you get a nicer flow. People often feel they get a nicer flow. But you may also get a text that lacks in precision, which is of course not what you want. So you have to check everything super carefully.
ANNA
And here I would say that don't fall asleep at the wheel. Meaning that if you just have one paragraph, you have to be super attentive and check everything so nothing changes. But, you know, if you just think, oh, this is good and you put in a bigger chunk of text, it's so easy just to lose track of what you're doing and you just think everything sounds good. So, well, you can use it, but you have to be super cautious. And I would say that some of the things you can use it for is brainstorming, like getting ideas or getting some feedback. But it may be better to ask for feedback than to get a rewrite.
LOVISA
Yeah, and of course to be able to evaluate this, you need to have some kind of background knowledge. You need to know that the sentence actually gets better and improved. You need to be able to see that yourself and be confident with that.
ALVIN
Yeah, I'd also agree with all of that. But I'd also add, this isn't actually unique to language models. I would say exactly the same thing with your human co-authors if you're writing an article, for example. Because you'll discover they'll also do weird little things like replace the word method with methodology because they think it means the same thing and so on. That sort of thing happens when you let people change texts in general.
ANNA
Yeah, and I would like to say that there are some tools that you can use. That, for example, Grammarly, the main function of Grammarly or InstaText. Because if you use those tools and not large language models, instead of getting a rewrite that's based on statistical likelihood, you get just suggestions. And those suggestions are based on grammatical rules or rules about concision, precision, level of style, for example. So there's a pretty big difference there. But I mean, you can use them, but I would not just go for the ChatGPT version just because it looks better on the surface. Can I use large language models to summarize texts? Because we all know that people are stressed out and having to read so many articles. So a lot of people seem to have taken the habit of using these to summarize.
LOVISA
Yes, and this is something we hear a lot about, that people use these tools to summarize text. So, can you?
ALVIN
I would strongly discourage doing that. Large language models, they can shorten texts but they kind of can't actually summarize them. And that might actually be hard to notice unless you read very closely what comes out of it. Because the problem is, if you give ChatGPT or whatever a long text and you say, summarize this for me, it will say, yes, of course. And then you will get something back that is short, contains many keywords from the longer text and that stylistically looks like a summary. Because it can do all of those things. It can recognize that these words occur here, so they should probably be there. It recognizes what a summary tends to look like. It doesn't actually understand what the original text is about, which means it doesn't understand what's significant. So it doesn't know what part to pick out and what parts it can throw away. So it's kind of just going to throw away things a bit at random and give you a shorter text, which might reflect the original text and it might not.
LOVISA
Yeah, and we've seen this many times, like how the summary is sometimes correct, sometimes it makes things up. It includes things that is not true, that is not written in the original. But even if the summary or the shortened text is correct, we've seen that it misses the core, the core of the original text. So the core is completely removed.
ANNA
Yeah, I've talked to so many people who have actually used these to summarize texts and they have been kind of happy about what it does. But I would say that what they do is that they ask for a summary first because they think it's kind of daunting to read a long research article. So they read the summary first and then they, after they know a little bit about the topic, they read the entire article and they feel a little bit more prepared. But I guess it's really important that you understand that you're not really getting a summary. But maybe you're just getting some kind of, you know, introduction to the field and then you have to read thinking about was this a correct summary.
ALVIN
Yeah, I'd also add the article probably has an abstract in it already. You can just read that.
LOVISA
Yeah, exactly. What is the point of these shortened texts when you have the abstract already?
ANNA
Another thing, this is quite related to the previous question, is about large language models that translate language like DeepL.
Is it okay to use DeepL? People think it works so well.
ALVIN
I would say sure, go ahead. Like I do think those are genuinely useful and I'm usually kind of negative otherwise about different types of language models. That said, the thing you have to keep at the back of your head is the sort of more even stronger version of what Lovisa said earlier. That how do you tell that a translation is any good? If I take a text in Finnish, translate to Swedish, well, I can look at the final product and see that, hey, this is probably a well-written text. But I don't know Finnish. I can't tell if it's actually a correct translation or if something terrible happened along the way.
LOVISA
But this is of course something you need to have a level of trust and you have to, if you have a translator, like a person who translates a text, you need to have a level of trust in that person as well.
ALVIN
Yeah, sure. But if you ask me to translate a text, you can get mad at me afterwards and possibly fire me if I do a bad job.
But what do you do when a language model screws up?
ANNA
Yeah, because I think that could be the consequence, right? That if we would write an email to someone who doesn't master Swedish or English and we just ask DeepL or a similar tool to translate the text, we could end up having major misunderstandings or even someone could become upset. Because I think also a professional translator would have some kind of understanding of the cultural context as well. Which of course a large language model probably doesn't.
ALVIN
It might to some extent because to some extent, culture actually is encoded in the statistical properties of language. But you don't know how much it understands.
ANNA
So I guess it's a little bit dangerous.
LOVISA
But still we all agree here that you need to have some kind of background knowledge to be able to evaluate what you get out and the outputs that you get.
ANNA
Yeah, and I think here too it's kind of easy to fall asleep at the wheel. Because you do see the output that's so great. So you tend to trust these tools more and more. But don't do that. You have to really know if the translation is okay or not.
Lovisa, there are some questions too about using AI to find articles, research articles. What do you think about that?
LOVISA
Yeah, and when ChatGPT came, that was something that libraries around the world had to deal with, that library patron came with questions about articles that they have received from ChatGPT, but that they couldn't find anywhere. They had tried Google Scholar, they had tried different databases, but they couldn't find these articles. And I think now I think most people do know that large language models, they can, so to speak, hallucinate articles or make articles up that do not exist. Even though ChatGPT has become better now. But we need to understand that it is a large language model and it's not a search engine, it's not a database.
ANNA
Yeah, I think I've found that one of the most useful ways of explaining large language models, when you talk about these fake references that were generated, which is, I mean, it is a fake reference in the sense that it doesn't work, but it doesn't exist. But the thing is that these have been generated based on statistical likelihood. So basically, if you ask about an article on this topic, you will get a title that ChatGPT or similar would deem likely that someone would have written. If they wrote about a topic, they could have written an article with this title. And if someone wrote an article with this title, it's likely that they would have written it with this author. They didn't, but it's likely. And it goes on like that.
ALVIN
I'd also add that you can integrate a database or a search engine into a large language model. There's a thing called RAG, retrieval augmented generation, for example, where you essentially, if you ask the model for, I don't know, an explanation of Newton's laws or something, it will check a database, find a text about Newton's laws, drop that into the context. window of the model. And what comes out will probably be true, like it's more likely that what you get is something true. But at the end, it doesn't remove the problem. Even when you do that sort of thing, it's still just fundamentally trying to figure out which words should follow which other words. It's just that hidden to you, it has some extra words that actually are true. And hopefully, it's going to sort of repeat the substance of those words. But still, it's not a physicist, it doesn't actually understand Newton's laws.
LOVISA
Yeah. And these tools that you are talking about are sometimes called AI search tool or large language models search tools. And there are several of those out there like illicit perplexity, and there are new tools coming all the time that works in the same way. And what usually happens is that you write a prompt into these tools, and then they work in a different way. Some of them translate that prompt that you write into a search query using so-called lexical search, the same traditional as you do in a traditional database. So it will pick up keywords and then search using those keywords. So the search is quite similar also to how you do it in a database. But then there are also tools that use semantic search, which is that it can understand the words in another way. It can understand the context of the words and so on. And what you usually get is the top five articles in these tools. And when you try different tools, you will realize that the top five articles are not the same in the different tools.
And it's not really transparent why you get those top five articles and why you don't get some other articles. So this is something that we usually say that you should be aware of when you're using these search tools that they are not transparent and they are not reproducible either. Like if you use the same prompt a week from now, you will probably get other articles. So you need to be careful to use these tools. You can of course use them as a starting point or as a complementary search, but you should probably not stick to one of these tools. You should probably try to use several of them or also do a traditional search in a traditional database. And when you're searching in a traditional database, what you get is that you get a list of articles. You don't get a summary of the different articles, but you get a list of all the articles that match the search query that you put in. And that is not the case when you're using these AI search tools.
ANNA
So I'm curious. So if you find a large language model that will give you an answer to the question that you ask it and you do get a lot of references in the text. And if I would then check up all those references and they seem okay, can I do that?
LOVISA
There is also a risk of confirmation bias, of course, that if you, for example, there are tools that that you can use when you write a text and then you can just use that text and trying to find a reference that will work to that text. But of course, then you don't know what's out there. You don't know what you're missing. You don't know. So that's something that you should also be aware of.
ANNA
It looks kind of like these are more reliable, but they're also a little bit more sneaky perhaps. In that sense, yeah.
ALVIN
Also, there's the simple problem that it might give you an answer if you're asking something and have a bunch of references to that answer. And if you take the time to actually read them, it turns out that no, they say no such thing whatsoever. That's quite common. And of course, that's not just limited to large language models. If you've ever done a literature search, we read articles, read the articles that the first article referred to and see how well each particular article actually managed to summarize what the other one was about. You notice human beings are also pretty bad at this.
LOVISA
But something I also think you should be aware of when you're using these AI search tools is to know where you're searching because some of these tools are integrated with, for example, Semantic Scholar, which is a so-called mega database. You search in a large, large database. And you can compare that to, for example, PubMed, which has been indexed with specific journals and they have been quality assessed that they can be indexed into PubMed. And that, of course, is also different from, for example, Google Scholar, which is an academic search engine that will just find academic articles or articles that look like academic articles, but that can be, for example, there was recently an article was published where they found lots of GPT fabricated journals in Google Scholar. So these articles were just made up. They did exist, but they were just written by a large language model.
ANNA
So the research behind it didn't exist.
LOVISA
Yes, exactly.
ALVIN
And I should say that's such a big topic in itself. I don't know, maybe we'll just make an episode about that one.
ANNA
There's another question linked to this. I found a blog post that's about my area of research and I was so intrigued. It mentions the article that produced the science but it doesn't give a proper reference and I haven't been able to find the reference. Could you help me with that?
LOVISA
Yes and this was a question that we did get at the library some time ago. We tried to find the article behind the blog post. We did some searches in Google Scholar, we did some searches in different databases but we couldn't find the article. And then we did eventually find it was very hidden that this web page was, it said, generated by AI or powered by AI, it said. So the blog post was probably completely made up but of course powered by AI can mean lots of different things. We don't really know what that means. Does it mean that it's a large language model that is just made this up and that the article does not exist or is it some kind of rag in this that the article does exist in some kind of sense? Most likely the article does not exist. But it was interesting because this also shows how hard it can be to actually evaluate when it comes to things generated by AI. Because even as a researcher it can be it can be hard to know that this is completely made up because it looks so good, it looks so true, it looks so like this could have been an article that was written.
ANNA
That is sneaky.
ALVIN
Yeah and unfortunately I think that's going to be more and more common because well this is an easy way to make money. Just take stuff from a language model and publish it somewhere.
LOVISA
Yeah exactly.
ANNA
And then those texts will be used to train new language models. That's a scary thought I think.
LOVISA
It is scary.
ALVIN
Yeah sometimes here people use the phrase Habsburg AI because the problem is that AIs they kind of have to be trained on actual human generated texts. If you feed AI with AI output you kind of get a form of inbreeding in a manner of speaking. So eventually they just start producing nonsense. And this is a huge problem for the AI companies now more or less irrespective of what we're talking about. Because if you do a Google picture search you're also going to get a bunch of stuff that doesn't exist because it's generated with GAI. So those are also going to well they'll be harder to train. It will be harder to find actual good data to train them with.
ANNA
Well data is the new gold. Is that what you normally say Lovisa?
LOVISA
Yes. So you should be careful what you put out there.
ANNA
Yeah. I was just going to say that we have a question about that too. Can I use generative AI? Is it safe or can my data be found and used by other people? And what about sensitive information?
LOVISA
We usually say that you should be careful about what data you're putting in there. You can also be aware that there are some settings. For example in ChatGPT there is a setting that says that you don't want the model to use the input that you give to train new models. But of course you should always be careful and maybe not trust this.
ALVIN
Here's where I would introduce a really good rule of thumb called the My Friend Bob rule. Nobody in Sweden is named Bob so I guess we'd call it Min Kompis Leif Regeln or something like that. The basic idea in a way is that if you want some quick way of figuring out whether it's a really really bad idea to do something using an AI. Just in your head try replacing AI with My Friend Bob. If I say I had some trouble getting started writing this so I asked my friend Bob for some ideas and he was pretty helpful I managed to get started. That's a reasonable thing to say. If I say I asked my friend Bob to diagnose this patient. That's probably professional malpractice and may literally be a crime. So don't do it.
ANNA
Yeah so you're also saying that secrets you wouldn't tell your friend Bob you shouldn't share with.
LOVISA
Yeah because you never know what he's going to do with it.
ANNA
No that that's correct. So we're kind of approaching another question. Can I use generative AI?
LOVISA
Yes and that depends on how you're using it of course. But when it comes to academia there are many journals now that have different kinds of policies how you should disclose the use of AI.
ANNA
Thank god. You remember in the beginning right where we would see ChatGPT as a co-author?
LOVISA
Yeah.
ANNA
So those guidelines, they are quite different still, aren't they?
LOVISA
They are. And some journals want you to write in the method section how you used generative AI. And some want you to have an acknowledgment. And some want you to have an AI disclosure. So it's very different. We haven't really figured it out.
ANNA
No, but I think we're getting there. We don't have these crazy things like having ChatGPT as a co-author or someone thanking the developers at OpenAI in their acknowledgment section. So we're getting somewhere here. And I think if you look at these different policies, at least, there are some things that they all have in common. Transparency. You have to be very clear about how you use these tools. And accountability. You're always responsible for everything in the text. And then I think also that they all seem to be a little bit restrictive on how much you can use generative AI because of course they don't want to publish articles that have been just generated by generative AI tools. And I think here too that now we talked about journals. But if you're a student or a doctoral student, you should check with your teachers. And I think that not everyone may have figured everything out yet. But we should try to be as transparent as possible and as curious as possible about this. And always ask.
And I would say for students I always recommend having some kind of AI reflection. So that it isn't just a statement of how you used AI but that there's always this element of reflection. How did you use AI? How did it work? How did it not work? What did you learn? Because you have to think about that all the time. Can you really say that you're fulfilling the criteria for your degree if you use generative AI in this way? And I think there too my friend Bob can be very useful. I mean you can ask your friend Bob to test out some ideas. But you can't ask your friend Bob to write and finish your assignment for you. Of course your teacher wouldn't be happy with that. But I do think it's very nice to have this reflection and this transparency there too. Because I also think that if we're super transparent about our use as students, we should not risk being accused of cheating. Because cheating requires some intent. So if you're transparent about your use, yeah you shouldn't be accused of cheating.
So I think that creates a safe space which you can then enforce as a teacher through encouraging open dialogues.
ALVIN
You kind of touched on a slightly larger problem there when you mentioned that you're responsible for what's in your articles. This has been a problem before large language models entered the picture. For a very long time in many fields there has been kind of a custom of gift authorship. That somehow I owe you for something so I add you as a co-author to my paper. Which you will see as a nice thing to do. And then it turns out that my paper is really bad. At which point you might feel that no, I haven't even read this paper. How can I be responsible for it? But the problem is that yeah, if you are an author, you're supposed to be able to stand behind every single word of the article.
ANNA
Yeah, I've seen retractions based on that very scenario that people didn't know that they were co-authors and weren't very happy about it. So my impression is that publishers are a little bit harsher on that now because they don't want drama like that.
ALVIN
So I'm not the one who receives most of these requests, but I have gotten a question about how can I use AI to analyze data? The short answer is don't use ChatGPT or any other language model to analyze anything whatsoever, it will end horribly. That said, you can do really interesting things with machine learning algorithms. Step one is to find a programmer who actually understands machine learning and explain to that person exactly what it is you want to do. And they can probably, hopefully, do something really useful. Do that before you have your data, don't do it after. And that's true in general, even if you just want to do a basic statistical analysis. Talk to a statistician before you get your data. There is even a quote I've had reason to give several times, which is by R.A. Fisher saying, to consult the statistician after an experiment is finished is often merely to ask him to conduct a post-mortem examination. He can perhaps say what the experiment died of." So that's important to keep in mind.
If you're in the situation that you have a bunch of data and you're now thinking about how can I analyze it, how can I analyze this, things have gone wrong. So that's my one question. Do you have any more?
LOVISA
Well, there are many more things to talk about, of course. Now we haven't talked about, for example, open science, open data when it comes to the age of large language models.
ALVIN
Yeah, and I think we should maybe start try to wrap up. But also, now we've been trying to answer people's questions, now I just want to check, do we have any questions of our own that we genuinely don't know, but we've been thinking about this?
LOVISA
There has been a huge hype around large language models lately, ever since ChatGPT. And I'm just thinking, are they here to stay or will we see kind of decline of them? What do you think? What is your thoughts on this?
ANNA
To me, I mean, yeah, they don't seem as hyped anymore. And I meet with a lot of students and they don't seem as excited about them. But they do seem to take them for granted and they do use them, often wisely, but they do seem to take them for granted. So I think the hype may be over, but I'm not sure that I think that large language models will disappear. What do you think, Alvin?
ALVIN
Kind of the same. Like I don't foresee ever saying large language models are like zeppelins, like they're gone now. I don't think that will happen. That said, I think they'll be used a lot more rarely because now all of these services are more or less free. That's not sustainable. OpenAI are burning money all the time. They lose money every time you use ChatGPT. At some point they'll have to stop doing that. They'll have to switch to some kind of subscription based model. And once you look at how expensive it is, I don't think you get so much use of ChatGPT that you will be willing to pay for it. Large language models will be used less and also because, well, they don't really have that much in the way of clearly defined use cases that you could put into, you know, actual industrial use. So they'll mostly just be things you play around with.
ANNA
And I guess there's also the environmental aspect that powering these machines takes so much electricity and cooling down these servers takes so much water. So it's not really sustainable.
LOVISA
And I also agree with both of you about the future of large language models. And I think when it comes to searching, I think what we will see more of is semantic search. And maybe not as much the large language models search tools, but we'll see.
ANNA
That's interesting.
ALVIN
Right. So I guess my question would be examinations.
Like for a really long time we have been telling students, write a thing and hand it in by Friday. And there's been a decent chance that the student actually wrote what they handed in. Today there is, well, now everybody can use just a large language model to write for them. So will we have to change our format for evaluating student performance entirely? Or do you think we can sort of adapt what we're already doing?
ANNA
Well, I think that's a wonderful question. And I do think some teachers were understandably scared when ChatGPT was launched. And they tried to just avoid the issue by giving oral exams or by having just exams with no internet access. But I don't think that's sustainable. I do think that we're going to have to teach our students to use generative AI responsibly. And I do also think that students need to be able to write. And another thing, I liked how you started this question, Alvin. Because I think for too long we have kind of equaled a well-written text with a passing or passed with distinction grade. And now we can't. And I think it's kind of reasonable that we can't. And I think this kind of forces us to ask questions such as what is really the purpose of going to university? What do you as a student have to show to your teachers that you know? What do your teachers need to understand in order to assess you in a fair way? So I think these questions that we haven't talked so much about, I think when we talk about generative AI, this is our chance to return to these super important questions about education.
LOVISA
I think that's a nice way to wrap this up. But because I also think this question about examination is something that when we started going out and talk to researchers and teachers as KI, it was the most common question that we got. This question about examination and how to deal with that.
ANNA
Yeah, and I think it's important to just note that so far, there are no AI detectors that are really reliable. Those that have been in use have been proven to over identify text as AI generated when the author is a non native speaker of English, which of course isn't fair. And also I don't know what it tells you that someone used generative AI to write the text. Maybe they used it in an okay way. It doesn't really tell you anything. And if we compare this to if you have a non plagiarism tool, anti plagiarism tool, I mean that gives you the possibility to compare text to something else. And you will have a clear record of, okay, this is exactly the same text. So you can assess it whereas there are no traces like that with generative AI. So it's a completely different situation.
ALVIN
Yeah, and I could add that I don't think there ever will be reliable AI detectors. The reason why I think that is a bit technical, so I'm just going to leave it out and say this is my bet about how the future will play out.
ANNA
A cliffhanger.
ALVIN
All right, do you have any like, I don't know, shout outs, like recommendations to other podcasts or papers you've read or blogs or whatever that you would like to direct listeners to that, hey, here's a good place to learn more about this topic?
LOVISA
When it comes to AI in searching, I would recommend, there are many things to recommend, but if I only choose one, I would recommend Arante's blog about searching. And he writes a lot about AI search tools and the problems with them and how you can use them in a responsible way. You don't have to, Anna. You don't have to.
ANNA
Alvin, I'm sure you have enough tips for all of us.
ALVIN
Yeah, I actually have lots of recommendations. So there will be a little Zotero library in the vicinity of wherever this podcast is uploaded where you can look up some things that we thought were relevant to look at. But off the top of my head, I would recommend a blog named Pivot to AI by Amy Castor and David Gerrard. David Gerrard is also the author of the book Attack of the 50-Float Blockchain, which is really good. Because that blog gives you a lot of the economic and social context to why the AI field currently looks the way it does. Because there are many things that kind of don't make sense if you only look at it from a technical point of view. This is going to sound weird, but in general, I recommend reading bitter tech bloggers who swear a lot if you want to understand the AI field. They're often the best source available and that's not a joke, that's just factually true.
ANNA
Yeah, well, I would say that my best advice would probably be to look at a reputable journal within your field. I look at Nature's homepage every now and then to see what they've written recently about generative AI. Which is interesting, not because they're experts, but it's interesting to see what concerns are hot right now. You know, what people are talking about right now in the field.
LOVISA
Yeah, I agree.
ALVIN
Okay, so then I guess we're done.
LOVISA
Yeah, do the outro, Alvin.
ALVIN
Yes, I'm outroing away. Thank you for listening to our FAQ on large language models. There will probably be more episodes about AI in the future, focusing on how the field of AI affects the library world. The podcast is made for the Karolinska Institutet Library and the responsible publisher is Miriam Nauri. I've been Alvin Gavel.
LOVISA
I'm Lovisa Liljegren.
ANNA
And I'm Anna Borgström.
ALVIN
You can find out more about us and the library at kib.ki.se. There you can also find other episodes of this podcast and information about upcoming episodes.
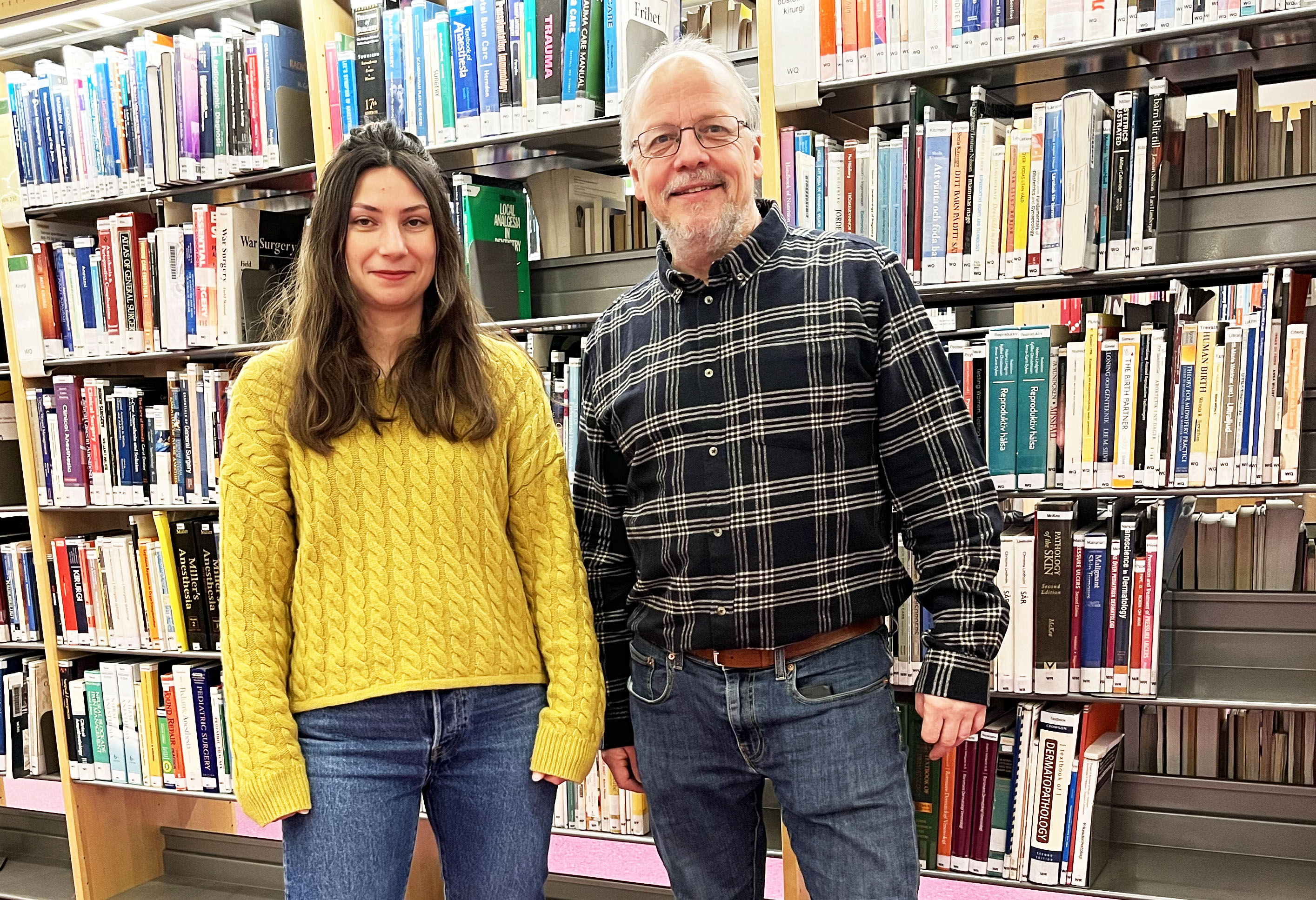
Pilot: We talk about systematic reviews with Wim
In the pilot episode, we meet Wim Grooten, associate professor, researcher and lecturer. We talk about systematic reviews, a form of literature review that aims to synthesise all available research or evidence in a defined area using a specific methodology.
- What is most difficult about writing a systematic review?
- Can AI be used in the process?
- Don't forget your PICO!
This episode is in Swedish.
If you would like us to get back to you, please submit your contact information in the form below along with your feeback.