Visualisation
In the sciences, visual material and visualisations can be used for a variety of purposes: to analyse and learn more about the data in question, to enrich research, or to communicate research data to others. This brief guide primarily focuses on the latter, the communicative aspects of visualising data. If you want to learn more, check out the University Library's open online course on visualisation, which contains in-depth material, case studies and practical exercises.
Why visualise?
To visualise means making visible the conditions or circumstances surrounding, for instance, a set of data. Your purpose in doing so might be either to continue analysing or exploring the data in pursuit of additional information or knowledge, or it might be to explain or communicate the knowledge or information that the data is generating. As always when it comes to scientific work, the quality as well as the methods used to collect the data will affect what kind of knowledge can be generated or communicated.
Visualisation is a powerful communicative tool. This is because visualisation leverages human perception, that is, our visual sense and the brain's ability to manage visual information, in order to interpret data easier, faster and better. Because visualisations are visual aids that can help free up working memory and effectively reduce cognitive load, visualisation can also be considered an assistive technology.
Audience and purpose
Audience and purpose are closely connected when it comes to visualising data. A simple (and somewhat simplistic) way to look at the connection between purpose and audience is to describe visualisation as existing on a scale between exploratory and explanatory visualisation. In some texts the terms discover and present are used instead of explore and explain to describe the separate ends of the scale, but the underlying meaning is the same.
Exploratory visualisation
Exploratory visualisations may, for example, consist of images in clinical practice, microscopy in laboratories, network analyses, epidemiological studies, and so forth.
- The target audience of an exploratory visualisation is generally someone with extensive knowledge of the data or research field in question. In other words, the intended audience for an exploratory visualisation has sufficient knowledge to explore, analyse and translate visual data into relevant information and knowledge. You yourself might be the intended audience of your visualisation, or the audience might be a group of colleagues with an equivalent level of expertise, or a clinician responsible for a patient's medical care.
- The purpose of an exploratory visualisation might be deciding what the next step in the research process should be, or the direction of a medical treatment, as an exploratory visualisation may help you understand data better, or in a new way.
Explanatory visualisation
Explanatory visualisations include, for instance, popular science communication or educational material.
- The target audience of an explanatory visualisation will be someone who lacks discipline-specific knowledge. Part of the work of any explanatory visualisation is communicating something relatively complex as effectively as possible to an audience lacking in-depth knowledge of the subject area.
- The purpose of an explanatory visualisation might be to convince decision-makers, to communicate the results of a study to the general public, to explain and justify a choice of treatment to patients, or to teach university students.
What makes a visualisation 'good'?
In his 1986 article, "Automating the design of graphical presentations of relational information", Jock Mackinlay describes graphical representations as a visual language, and suggests two design criteria whereby one can formulate and evaluate sentences uttered using this particular language:
- Expressiveness: A set of facts is expressible in a visual language if the sentences (i.e. the visualisations) in the language express all the facts in the set of data, and only the facts in the data.
- Effectiveness: A visualisation is more effective than another visualisation if the information conveyed by one visualisation is more readily perceived than the information in the other visualisation.
In short, while expressiveness is about what data that is being described, effectiveness is about how that data is represented. For a visualisation to be considered 'good' it would need to be both truthful and accessible.
The two criteria also affect each other, that is, the content of the data affects how it can and should be presented. An important concept to consider here is encoding, which describes the process of translating information into a graphic representation. To take a simple example; drawing 50 percent as one half of a circle in a pie chart is the act of visually coding information. When doing so, keep in mind that there are often several possible visual variables that you may use (for example colour, position, area, etc), and that some ways of encoding data are easier for people to interpret accurately than others.
Another aspect to take into account is that different types of charts have different strengths and weaknesses, and can be used to convey different aspects of any data set. For instance, the use of pie charts is often criticised; they aren't regarded as exact enough; it's difficult to compare the size of the pie slices, etc. These criticisms aren't without merit, but a pie chart also has some advantages. For instance, if the slices are few and clearly distinguishable, we tend to decode them very quickly.
Recommended reading
Franconeri, Padilla, Shah, Zacks & Hullman. (2021). The Science of Visual Data Communication: What Works, Psychological Science in the Public Interest, 22(3), 110-161. DOI: 10.1177/15291006211051956.
This article is available via the University Library. It provides an overview of the theoretical basis and a summary of guidelines and key principles for visual data communication.
Learn more
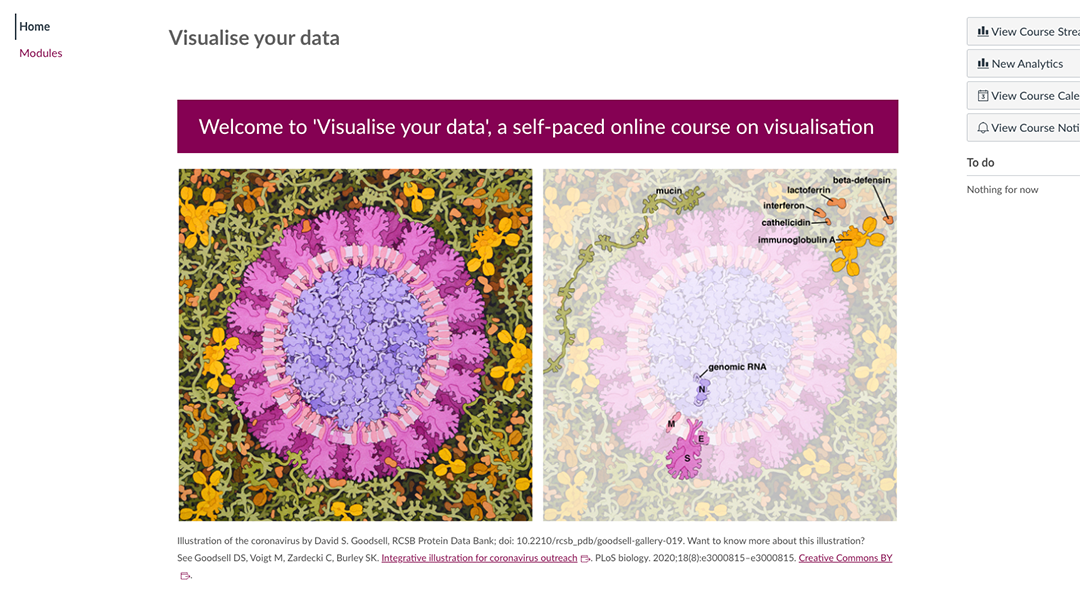
Self-paced course on visualisation
The University Library’s free, open online course on research data visualisation provides you with a basic introduction to the field. Through case studies and practical exercises, you will learn to graphically represent and communicate data in an effective and accessible way.
Resources for visualisation
There are many different resources for creating visualisations. Here we address some of these and their strengths.
Graphical abstracts
Being able to create a visual summary of the key findings of an article is becoming more and more important. The summary is usually comprised of a single image designed to help the reader to quickly identify the relevance of the written material.
If you would like us to get back to you, please submit your contact information in the form below along with your feeback.